Can Machine Learning Help Give Boost to Flagging Healthcare?
According to the World Health Organization, more people than ever are living into their 60s and older; this population will comprise 20 percent of the world’s population in 2050.
Our longevity can be attributed to several factors, but the outsized role of advances in health care, although unquantifiable as a single factor, is undeniable.
If only healthcare could be provided efficiently to our over-surviving populace. It is no secret, however, that the industry is facing enormous challenges.
Shortage of Professionals
According to the Association of American Medical Colleges, by 2025, there will be a shortfall of between 14,900 and 35,600 primary care physicians; the shortage of non-primary care specialists is projected to reach anywhere from 37,400 to 60,300 by that same year. Updated 2018 data from the AAMC indicates that by 2030, the shortage will reach a whopping 120,000 overall (a shortfall of 14,800 to 49, 300 primary care providers, and 33,800 to 72,700 non-primary care providers).
Along with this shortage, myriad issues continue to derail the provision of quality healthcare including:
- Provider burnout, which is strongly associated with medical errors.
- Disparate electronic health records impede data-sharing of vital information such as patient history and medication profile
- Drug and medical supply shortages
- Resurgent diseases
- Antibiotic resistance
- Administrative inefficiency and issues with insurance reimbursement, claim-processing
- Rising costs of care and medications
Despite the incredible connectivity afforded by technology in the 20th and 21st centuries and near-daily medical advances, few will argue: The U.S. healthcare system is broken, consistently ranking as the most expensive and the least efficient in countless reports.
Providers and their staffs are inundated with paperwork, growing demands to see more patients in less time, rising costs of malpractice insurance – all while striving to stay abreast of breaking studies and new treatment protocols.
Could providers use … an extra set of processors? Could the entire industry?
Artificial Intelligence: Medicine, Reimagined
The 21st century has brought about the growing use of technologies that use artificial intelligence (AI) to complete tasks and reasoning once performed by humans, including providers. Britannica defines AI as “the ability of a digital computer or computer-controlled robot to perform tasks commonly associated with intelligent beings.”
Granted, there is a strangeness, even creepiness to devices that are programmed to reason, discover meaning, generalize, and learn from experience, qualities that may seem amazingly – or alarmingly – human. But in one sense, AI is nothing new. The founding father of AI and modern cognitive science was British mathematician and logician Alan Turing. A codebreaker during World War II, Turing’s post-war research focused on the parallels between computers and the human brain. The scientist considered the cortex to be an “unorganized machine” that could be trained to be organized into “a universal machine or something like it.”
The term artificial intelligence, however, was not coined until a few years later, in 1955 by Turing contemporary John McCarthy, a mathematics professor at Dartmouth College. McCarthy defined AI as the “science and engineering of making intelligent machines.” Shortly thereafter, McCarthy convened the first AI conference and subsequently created one of the first computer languages, LISP.
It took a while (35 to 40 years) for computers to be a staple of providers’ practices, and a ubiquitous item in patients’ purses and back pockets. But once computers became commonplace in our society, AI-powered computers and robots were the next logical step, right?
But not so fast.
These new-fangled machines bring unwelcome anxieties and fears. Will human beings be displaced and replaced by these unnerving inventions? Will AI be running the world? What will people do all day when machines are doing everybody’s job? Are providers on the brink of extinction? These questions are justified, and the feelings are natural. But we’ve been here before.
Truth Stranger Than Fiction?
Culturally, AI has long been the subject of dystopian sci-fi movies and a recurring source of dread, foreboding, and even hysteria. Nearly 100 years ago, in the 1927 classic “Metropolis” the robot Maria mixed it up in a futuristic, mechanized society dominated by power, manipulation, and social engineering. That film came on the heels of a 1930s America mired in the throes of the Great Depression, massive automation, and culture-altering mechanization spurred “robot hysteria.”
And the trend continued. In 1968, we had the epic showdown between rogue computer HAL 9000 and astronauts in “2001: A Space Odyssey“, The Terminator fighting killer machines in the 1980s, and the manipulative and murderous robot Ava in 2014’s “Ex Machina.” For nearly a hundred years, our society has had a love-hate relationship with machines that think and act as humans do.
These fears bear examination and warrant validation; for providers, the influx of new AI-driven machines may seem to supplant physicians’ expertise, experience and mere existence. Will AI take over the roles of diagnosing patients and creating treatment plans? Who will run our practices, clinics, hospitals? Robots? Computers?
What exactly are these machines doing?
AI: Processes That Are Human-Like, But Not Human
Although the idea of smart computers that “learn” without our permission may seem alien, most of us already use AI in our everyday lives. Assistants such as Apple’s Siri, Amazon’s Alexa and other “personal assistants” may seem innocuous enough, but the truth is these and similar devices use machine-learning to better predict and understand our natural-language questions, interpret our speech patterns, and anticipate our future needs and habits.
Netflix, for example, analyzes, in a flash, billions of records to suggest films a consumer might like (and order or purchase) based on that person’s previous choices and ratings of films. Google very quickly anticipates websites of interest targeted to each user, Amazon has long been using – and constantly refining – “transactional” AI algorithms to keep track of individual’s buying habits to assess, predict and suggest purchasing behavior.
How do they do it? Welcome to machine learning (ML is one of the most common types of AI used in the medical field). ML is the application of statistical models to data using computers. Machines that use ML do not “think” independently; rather, they are programmed with algorithms – a set of criterion that establish a process for recognizing patterns and solving problems.
The jury is out on whether consumer-level leveraging of AI to suggest where we should eat or who we should link with on LinkedIn is useful. But there are other ways that machine learning is used that has positive global effects.
Energy giant BP uses the technology in sites worldwide to maximize the use of gas and oil production, so it is safe and reliable –something we all want when we start our cars every day. Its data is also analyzed by engineers and scientists to plan for future energy needs and develop new model and energy sources. In a world with limited resources and a burgeoning global population, AI undoubtedly helps to keep prices low and oil and gas available.
GE Power similarly uses machine learning combined with big data and the internet of things to optimize operations, maintain equipment and attempt to develop digital power plants. We all like it when we turn a switch and the lights go on and take for granted that the H tap will keep hot water flowing into our homes.
Many financial giants use AI to predict market trends, allowing investors and retirement funds to allocate money wisely, as well as cut down on fraud and other financial crimes, which can drop the price of these types of services for individuals and employers who rely on their data for building retirement funds.
What Can You Do for Me, Mr. Roboto?
The innovations in medicine and related industries in 21st century has brought about an astounding array of new medications, advances in imaging, and reimagined treatment protocols, all at a break-neck speed.
How is a provider to keep up with all these changes, the endless journal studies and clinical trial data, new treatment options counter-balanced by black-box warnings, abrupt changes in long-held treatment protocols, reporting requirements, and harbingers of disease-outbreaks?
Not to mention the ever-increasing pressures that healthcare entails.
- Data, data, data. AI-driven machines and their algorithms quickly incorporate myriad variables across a broad array of complex screening and diagnostic information and data. The information is processed to predict future events (e.g., what treatment protocol for disease X in population Y has led to the best outcomes based on previous cases and outcomes.) AI systems constantly update as new data are added, learning new associations and refining their predictive power in real time. Machine learning can help to reinforce currently successful interventions and – perhaps more importantly – reveal new trends and raise new questions based on trends in data.Ostensibly, once compatible global AI systems are in place, providers will have access to data gleaned from every patient treated for any disorder in every country, plus breaking medical discoveries and clinical trials outcomes, all housed within AI-driven machines and their algorithms. The incidence of medical errors and ineffective care caused by incomplete patient electronic health data, missed treatment opportunities, and misdiagnoses will be greatly reduced, perhaps even eliminated.
- Eliminating bias. Another quality of AI is the blind nature of its data processing. Clinicians gain valuable knowledge through experience over time based past patient cases. This knowledge, however, can introduce prejudice to the decision-making process, and lead to misdiagnosis, treatment based on old data, and missed opportunities for more current approaches. If done correctly use of AI can eliminate these potential pitfalls, as clinical recommendations are not based on a priori assumptions, but rather on constantly updated medical data drawn from myriad sources.
(CAUTION: Biased data can introduce the very biases you are trying to avoid.) - Building Patient Profiles/Predicting Outcomes. Recommending an effective treatment plan is predicated on certain provider tasks such as taking the patient’s history, performing a physical exam, and incorporating information from established research (all of which are vital in screening and diagnosis). The goal to deliver a specific outcome requires planning and implementation of said plan (actions needed for treatment and monitoring). AI computers can quickly “crunch” complex data from the physical exam, lab results, and extant study data to match thousands and even millions of global data sets to predict future events (the best treatment plan for the desired outcome), in a matter of minutes or even seconds.
Growing Support for AI in Medicine
AI is already a part of standard practice in many medical networks; data show that intelligent machines are saving lives. China is using an AI program called Infervision to keep pace with reviewing the annual 1.4 billion lung CT scans ordered to detect early signs of cancer. The application has proven successful in addressing the country’s radiologist shortage and provider fatigue (and medical errors) associated with reading endless scans. Infervision is programmed to teach its algorithms to diagnose cancer more efficiently.
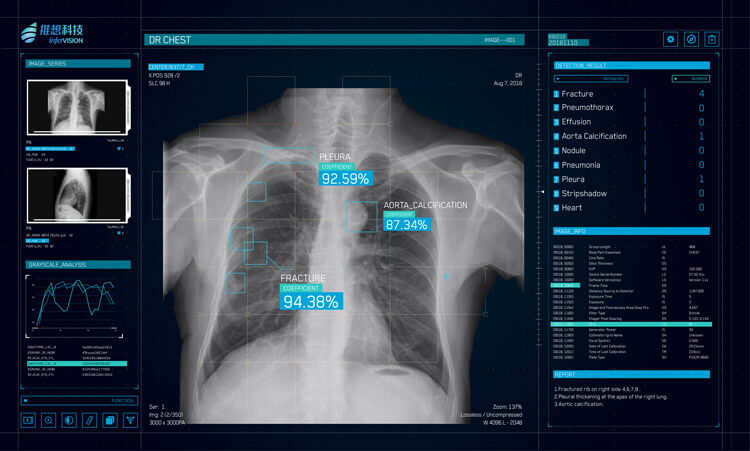
The University of North Carolina is using AI from IBM to comb through data such as discharge summaries, doctors’ notes and registration forms to identify high-risk patients and formulate prevention care plans for them. Google’s DeepMind initiative aims to mimic the brain’s thought processes to reduce time needed for diagnosis and formulating treatment plans. The implementation of DeepMind at the University College of London to quickly assess brain images and recommend appropriate, life-saving radiotherapy treatments.
But not every AI-driven medical application has been a success.
IBM’s AI-driven machine Watson (whose original claim to fame was beating human contestants at “Jeopardy” on prime-time TV), was hyped as a state-of-the-art personalized cancer treatment genius to millions of patients and providers around the world. In 2012, MD Anderson Cancer Center at the University of Texas paid Big Blue millions to partner in bringing Watson into the fold. In 2017, however, the university withdrew from the partnership. The main problems, although not clearly articulated by MD Anderson, may have been issues with procurement, cost overruns and delays. In addition, expectations rose to unrealistic levels due to publicity and hype.
Authors of a blog published in “Health Affairs” who commented on Watson’s failure noted that AI was no replacement for human involvement in clinical care; machines and robots are not poised to take on all the reasoning and complex tasks inherent in health care. They did concede, however, that AI is suited for reducing time spent on resource-intensive tasks.
Although Watson was not yet ready for prime time, the authors noted, “… carefully examining and evaluating opportunities to automate tasks and augment decisions with machine learning can quickly yield benefits in everyday care. Furthermore, by taking a practical approach to evaluating and adopting machine learning, health systems can improve patient care today, while preparing for future innovations.”
Don’t Let the AI Train Pass You By
The AI marketplace is exploding; according to a recent analysis by Accenture, AI will grow to a 6.6 billion industry by 2021; new health care AI initiatives are cropping up everywhere and investors are being encouraged to invest. This bodes well for providers; dollars invested in AI health care will assure that current applications are expanded, extant programs (such as Watson) will receive the tweaking and refinement they need, and that new AI opportunities are funded.
AI could hold the keys to improving our beleaguered healthcare system in ways that were unimaginable a couple of decades ago. Projections indicate that insurance companies could save up to $7 billion over 18 months by using AI-driven technologies to streamline administrative process. Several insurers are already using AI chatbots to answer simple patient questions and predict emergency-room visits.
Providers could similarly use AI for administrative tasks, freeing up staff for more patient-oriented encounters, expediting insurance reimbursement, and affecting referrals. That is in addition to the myriad care-related support function that AI can currently provide; many more are in the works and will be rolled out in the upcoming months, years, and decades.
Skepticism is natural, and thorough vetting is necessary to ensure that Silicon Valley and other Big Tech promises don’t override peer-reviewed research on the efficacy of AI applications; consistent testing and feedback from a wide array of providers in a variety of disciplines; and appropriate rollout to ensure the safety, accuracy and applicability of AI in medicine.
The robots and machines are becoming more powerful. No worries — there will always be a doctor in the house, and, like the new technology, she’ll be better than ever.
Lise Millay Stevens, M.A., is a contract medical communications specialist who has served at the New York City Department of Health & Mental Hygiene (Deputy Director of Publications); at the American Medical Association (managing editor of five JAMA journals) and then senior press associate and editorial manager of Neurology. Lise is a member of the American Medical Writers’ Association (past-president, Chicago), the New York Press Club, and the Society of Professionals Journalists.