How To Launch a Data Analytics Strategy
Creating a data analytics strategy can feel overwhelming, and many of them end up failing before they get off the ground. Here’s what to do to ensure your project is focused, worthwhile, and highly successful.
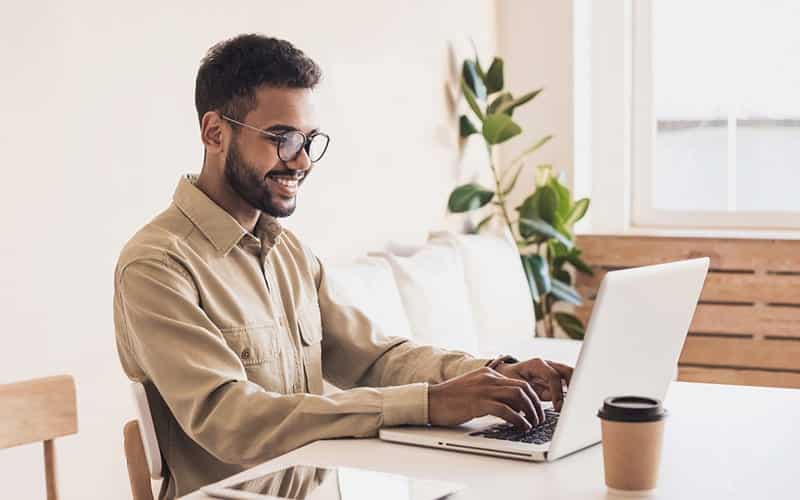
Becoming data aware is aspirational for all companies, but can be both a technical and cultural challenge. It is a journey and as such the starting point can vary. Some companies start with top down edicts that are too widely defined and end up bumping up against the organizational structure in the middle or bottom of an organization.
This potential resistance shouldn’t stop you from starting today. Insights from data analytics compound over time, which means that the sooner you act on your strategy, the sooner you can see real results from data-backed decisions.
We have seen successful launches of data analytics in an organization when they start small, focus around one specific impactful problem, and create value around it by doing the following three things:
- Open up the access to all pertinent data related to the problem you’ve defined and have decided to focus on.
- Encourage cross-functional collaboration in order to design and execute on that solution. Main functions needed for analytics include data engineering, data science, LOB analysts and management. Analytics is a team sport.
- Make technology decisions that accelerate the collaboration in design and delivery of the solution.
Data Analytics Strategy in Context with AI-Powered Search
Let’s use AI-powered search in the digital workplace to put these three keys into context.
Search is becoming more and more a mission spiritual application in the digital space. The whole point of digital assets and information is to be easily findable and discoverable. The more intelligent it is, the more impactful for customers or employees. It is a narrow enough field that it can be executed on, without a full overhaul of the organizational structure, which makes it the perfect problem to focus on when implementing a new data analytics strategy.
A. Open Up the Data: In a workplace information retrieval scenario, all the data sources need to be federated into the search engine, augmented and curated with the intelligence needed for relevant retrieval. Once the engine is deployed, search behavior data needs to be organized and used to continually improve the search relevancy.
The pool of data employees have access to, the business goals, and the people actually using the data is ever-changing. For example, a company like SAS with 16,000 global employees and over 9 million documents, can’t continue to improve search relevancy if they only have a frozen in time snapshot of how employees are accessing information. By opening up the data, project leaders can learn how the engine needs to be tweaked and improved over time.
B. Collaboration: Most organizations encounter multiple barriers to cross-team collaboration, including obstacles in processes, hierarchies, personalities, and culture in general. The best process, in theory, enables the type of iteration and back and forth where data scientists deliver the artifacts (models) that search developers can integrate, quickly test in the query experience, and if needed loop them back to the drawing board for refinement.
Most data analytics projects fail because of a lack of integration and collaboration. It’s less because teams don’t have the requisite knowledge, it’s more because there’s a lack of alignment on goals, expectations and processes.
C. Choosing Technology: Pick a search platform that accelerates data ingestion, imbeds intelligence and scales for operations. This efficiency will allow teams to spend most of their time improving the business outcomes, instead of the opposite: integrating inefficient process and technology hand-offs and minimizing the learning curves at each step.
For example, data scientists do not need to learn operational aspects of model deployment, and operations folks do not have to recode model packages or understand in full detail what they do. An AI-Powered engine should allow a seamless transition of model packages from data scientists into model deployment via immutable instances of that model that can be easily managed and scaled as needed by operations teams.
Selling Your Boss on Why This Approach Is Successful
Success breeds success and time-to-value tends to be one of the measures of success in data analytics projects. Starting small with a targeted application as described above increases your chances of success. Here’s why:
- Smaller organizational scope gives you more control and agility
- More control and agility allows for better planning and iteration
- Better planning leads to better and faster execution
- Better execution leads to better and faster business outcomes
One of the main learning curves for organizations is not only what to work on, but when you decide on what to work on, “how” you organize to design and execute. That can be learned by starting small and targeted and then scaling to the rest of the organization and use cases.
Hire T-Shaped Individuals
I have worked with over 400 enterprise analytics projects. Despite the fact that we hear that data scientists are in short supply, in my experience people that are able to organize and run cross-functional analytics projects are the true shortage in organizations.
These are “T-Shaped” individuals that have a blend of technology, business and AI knowledge with organizational skills that allow them to influence without authority and effectively organize and run these projects in the organizations. This profile is what McKinsey calls Analytics Translators. This skills set is hard to find because it needs to develop in time. Companies that develop, foster and grow this type of talent are the ones that will be successful in running impactful analytics projects.
Focused Start, Major Impact
Your leadership may be ready to completely overhaul every process in the organization. Pumping the brakes and focusing on one problem at a time is the best way to start. It’s a major undertaking but will have a serious positive impact on the bottom line. In the case of our AI-powered search example, that could mean faster decision-making from employees and happier teams that can find information at their exact moment of need.
Ready to build a data analytics strategy for your organization? Contact us today.
LEARN MORE
Contact us today to learn how Lucidworks can help your team create powerful search and discovery applications for your customers and employees.