SolutionsInnovation, expertise, and AI that solves your biggest business challenges.Why settle for average? Take advantage of AI-powered business solutions that transform your data into a strategic advantage.
Get a DemoTap into enterprise search and discovery that drives unprecedented growth.
We deliver knowledge management, customer experience, and customer service solutions—all powered by intelligent data acquisition, advanced AI, and expert services… however you choose to deploy.
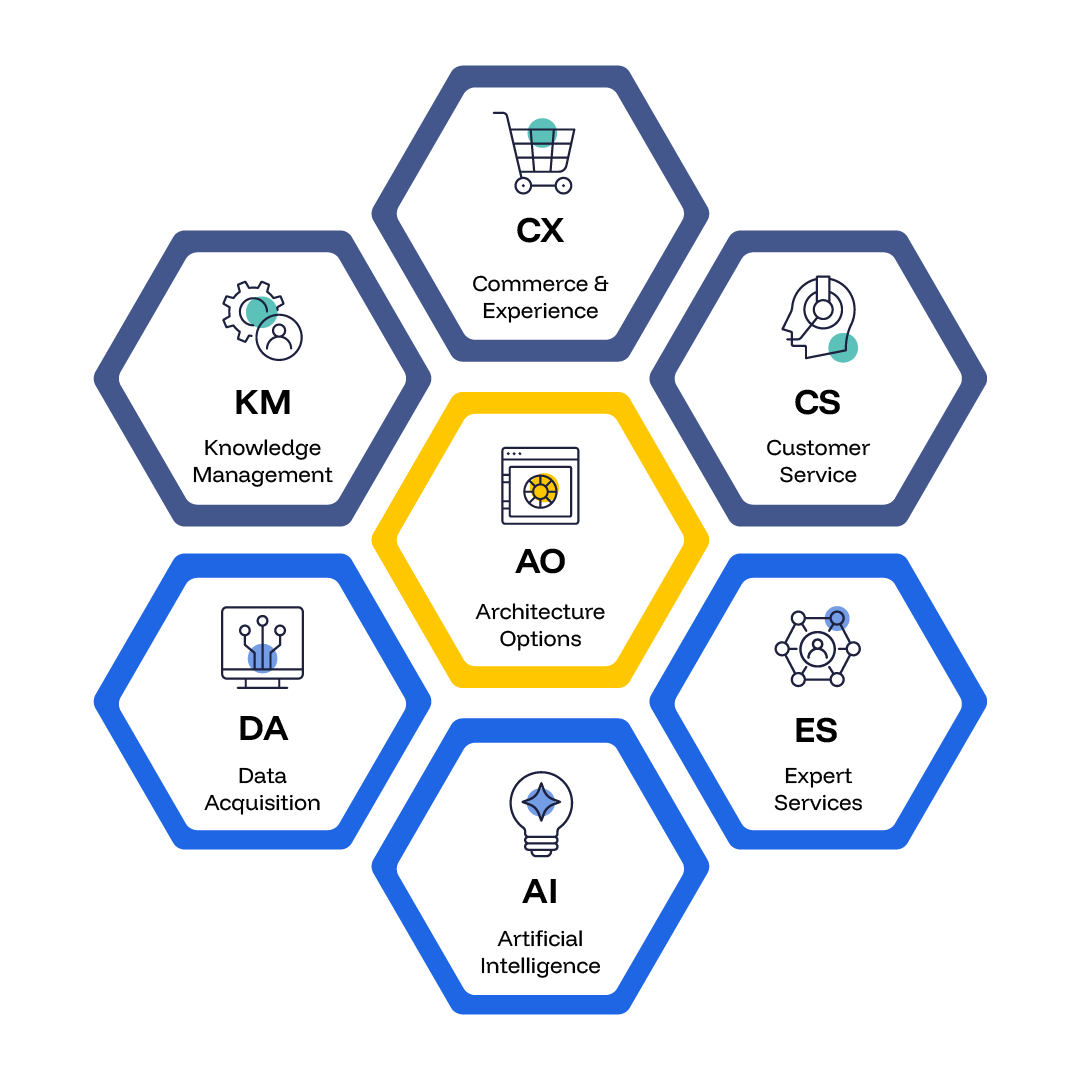
Transform your business with AI-powered search and discovery experiences.
Knowledge Management
Stop letting valuable information get lost. Our data-driven decision making solutions create dynamic knowledge systems that connect the right content to the right people, while automating curation to keep critical information current and accessible.
Client Success Offerings (CSO)
Turn great technology into real business results with our dedicated support team. Our AI-powered business solutions are essential—but so are the people who ensure your search and discovery tools deliver measurable impact.
Accelerate Your SuccessCustomer Service and Support
Give customers better help with intelligent automation solutions that resolve issues faster. From AI-powered chatbots to smart knowledge bases and automated ticket routing, we help your support team focus on what matters most.
Elevate Your SupportCore Packages
Get up and running quickly with pre-built templates designed for B2B and B2C organizations. These packages come with expert guidance to help you transform your business with AI that delivers measurable results fast.
Start Seeing ResultsSearch
Make finding information effortless with enterprise AI applications for search. Our technology understands context and meaning beyond keywords, learning from user behavior to deliver increasingly relevant results that match true intent.
Discover What’s PossibleCommerce
Transform online shopping with AI that understands what customers actually want. Deliver personalized recommendations, create eye-catching displays that drive sales, and use real-time shopper insights to make smarter merchandising decisions.
Boost Your ConversionsFrom challenges to triumph: Solutions that deliver.
Tailored Solutions
Every business has its own unique set of challenges. That’s why we’ve created a comprehensive suite of enterprise AI applications designed to solve real problems you face every day.
Practical Results
Whether you’re looking to boost online sales, improve customer support, or help employees find the information they need, our solutions deliver measurable results, not just flashy tech.
Holistic Approach
Our approach to business process optimization with AI examines your entire workflow—identifying bottlenecks and where intelligent automation solutions can make your team more effective.
How businesses put our enterprise AI applications to work.
Expert Services
We're with you every step of the way.
We support you through every part of your journey, a distinctive advantage of working with Lucidworks. Our global expert teams advise in search and digital program design, relevance improvement and optimization, B2B and B2C ROI, and full lifecycle implementation and support. Technology is essential, but so are the people who partner with you for your success.
Check Out Support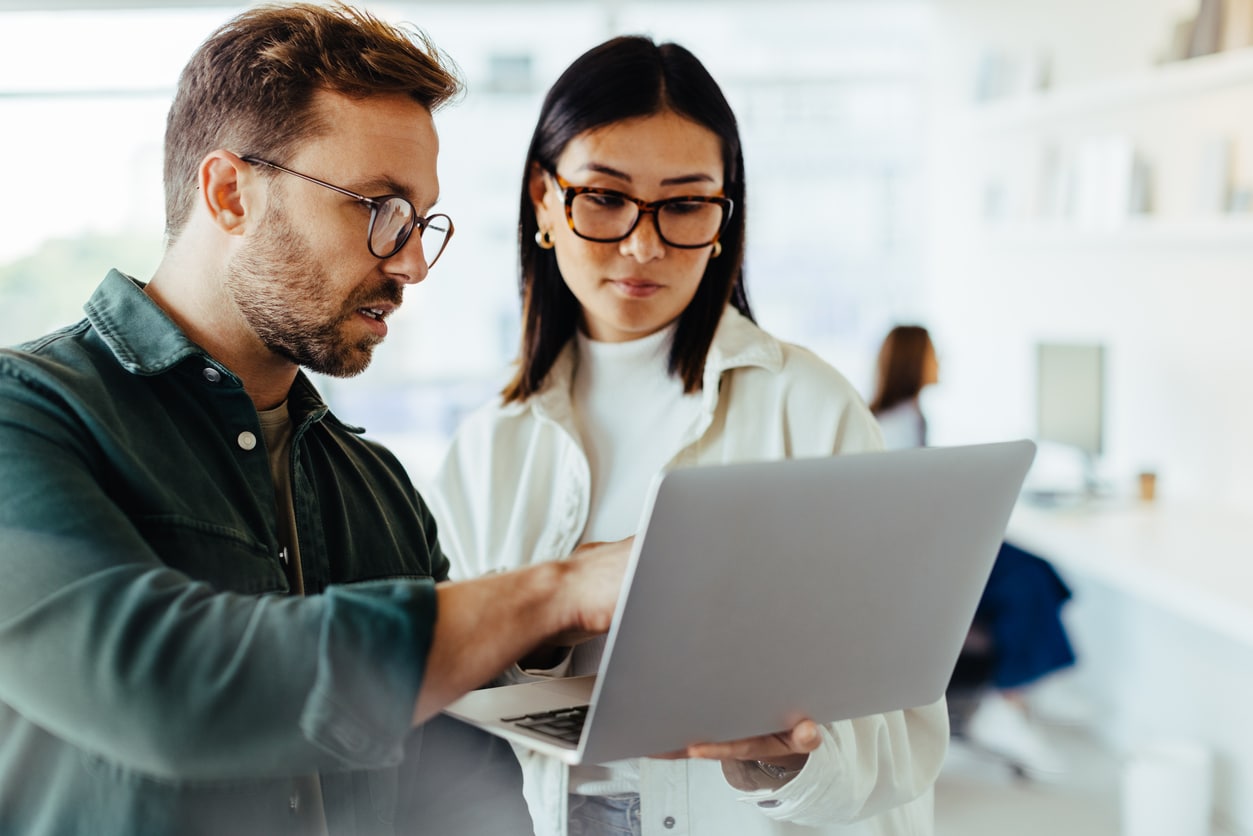
"Lucidworks has revolutionized the way we approach search and discovery."
David Lee, VP of Technology
Forrester's Total Economic Impact study shows our AI-powered business solutions deliver a remarkable 391% ROI in just three years, with customers breaking even in less than six months.
See the NumbersREI transformed their outdoor gear discovery with our solutions, creating personalized search experiences that significantly boosted revenue during their busiest seasons.
Explore Their SuccessLenovo implemented our enterprise AI applications across 180 markets, resulting in a 95% increase in search-driven revenue and 55% improvement in result relevance.
Watch Their Story