5 Things to Think About Before Pursuing a Gen AI Search Project
When it comes to Gen AI search projects for knowledge management, avoid costly mistakes with these 5 tips.
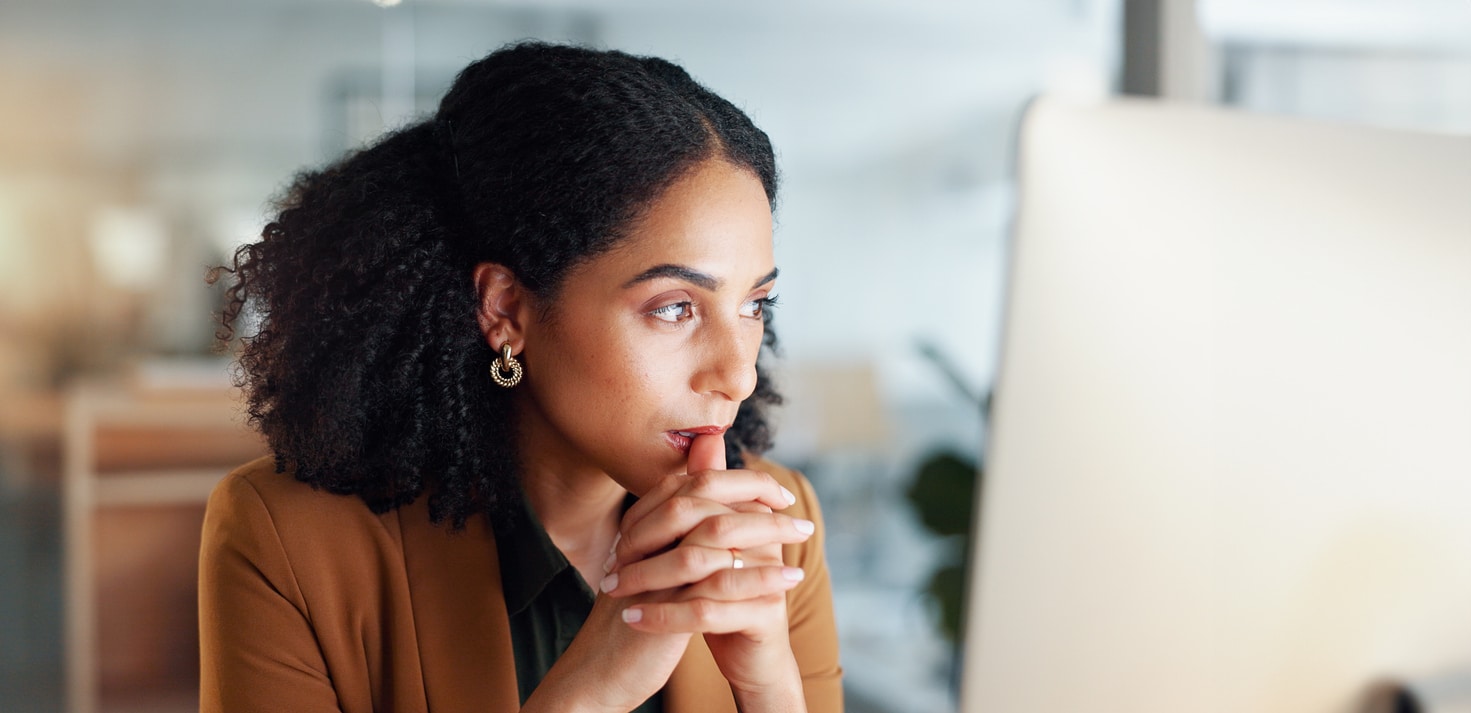
The emergence of generative AI (Gen AI) is transforming the way we interact with information, promising more intuitive, conversational, and real-time interactions. But while the potential is undeniable, it’s not always the most practical or cost-effective solution for every business challenge.
At Lucidworks, our experts have been immersed in the AI landscape for years. We’re excited about the possibilities of Gen AI search use cases, but we also know it’s not a one-size-fits-all answer. As Phil Ryan, our VP of Architecture & Technical Strategy, wisely notes, “There’s a lot of elements in this system, and you don’t need Gen AI for every piece.”
This article cuts through the hype to offer a pragmatic roadmap for leveraging Gen AI in your organization. We’ll explore how to maximize its value, mitigate risks, and avoid unnecessary costs. You’ll discover strategies for improving information retrieval, enhancing knowledge work, and creating a more intuitive search experience — all while keeping in mind that sometimes, simpler solutions can be just as effective.
Whether you’re eager to dive into Gen AI or simply curious about its potential, this article will provide valuable insights to guide your decision-making. Keep reading or watch our webinar to learn more:
Here’s what we’ll cover:
- How generative AI models like large language models (LLMs) can improve information retrieval, but with careful consideration of risk.
- How offline analysis of queries and data can enhance AI model performance over time.
- Key factors when implementing generative AI, like selecting models, integrating data sources, and measuring success.
- The importance of cost, flexibility, and the ability to handle data variability in generative AI solutions.
- Techniques to help generative models better understand large, complex documents and data sources.
Takeaway #1: Generative AI Can Improve Search, But Risks Must Be Considered
While the potential of Gen AI is immense, it’s essential to carefully assess the risks and implications of incorrect answers. User trust is paramount, and inaccurate information can quickly erode it. Start by identifying use cases with high information friction (areas where users struggle to find what they need) but low risk if the AI gets it wrong. Experiment early and often to validate the model’s performance in your specific context, and always be transparent with users about when they’re interacting with AI-generated content.
Takeaway #2: Offline Analysis Improves AI Performance Over Time
Analyzing past queries and data offline is a powerful way to help AI models learn and improve. This practice not only leads to more accurate responses but also generates valuable test cases for ongoing validation and quantitative measurement of model improvements. This can involve fine-tuning the model to better understand your domain-specific language or identifying gaps in your knowledge base. By continuously iterating and refining your AI model offline, you can ensure it delivers increasingly relevant and accurate results over time.
Takeaway #3: Select Models, Integrate Data, and Measure Success
Successful implementation of generative AI involves several key steps. Choosing the right model type for your specific needs is essential. Integrating disparate data sources effectively ensures that the AI has a comprehensive knowledge base to draw from. Finally, tracking key metrics allows you to gauge the impact of your AI implementation and make data-driven improvements. Remember, the “best” model today may not be the best tomorrow, so prioritize flexibility in your architecture and don’t get locked into a single solution.
Takeaway #4: Consider Cost, Flexibility, and Data Variability
Given the rapid pace of AI advancements, cost-effective and flexible solutions are a must. Models should be adaptable to the ever-changing AI landscape. Additionally, they must be able to handle inconsistencies and variability in unstructured data sources, ensuring that the AI can extract valuable insights even from messy data. While the cost of Gen AI is a factor, don’t let it be the sole deciding factor. Weigh the potential value against the cost, and explore open-source models if budget is a concern.
Takeaway #5: Techniques for Complex Documents and Sources
To truly understand and use the vast amounts of information contained in large, complex documents and data sources, specialized techniques are often necessary. Semantic chunking breaks down content into meaningful units, making it easier for the AI to process. FAQ extraction allows the AI to quickly provide answers to common questions. Embeddings, which represent words or phrases as numerical vectors, enable the AI to understand the relationships between different pieces of information. For messy data like Slack threads or discussion forums, consider using LLMs to generate FAQs, extracting the key insights and questions from the conversation.
Gen AI Is Not One-Size-Fits-All
Generative AI has the potential to transform how we search for and interact with information. However, it’s not a one-size-fits-all solution. As Phil Ryan wisely said, “…there’s a lot of elements in this system, and you don’t need Gen AI for every piece.” By carefully considering the factors outlined in this article, you can leverage Gen AI to power the next generation of search and knowledge work, without overspending or overcomplicating your solutions.
Sign up for our newsletter to stay up-to-date on the latest trends and insights in AI and search technology.
Best of the Month. Straight to Your Inbox!
Dive into the best content with our monthly Roundup Newsletter!
Each month, we handpick the top stories, insights, and updates to keep you in the know.