Generative AI Unlocks the Door to Unstructured Enterprise Data
Gen AI has CIOs rethinking data sources and reexamining their unstructured data.
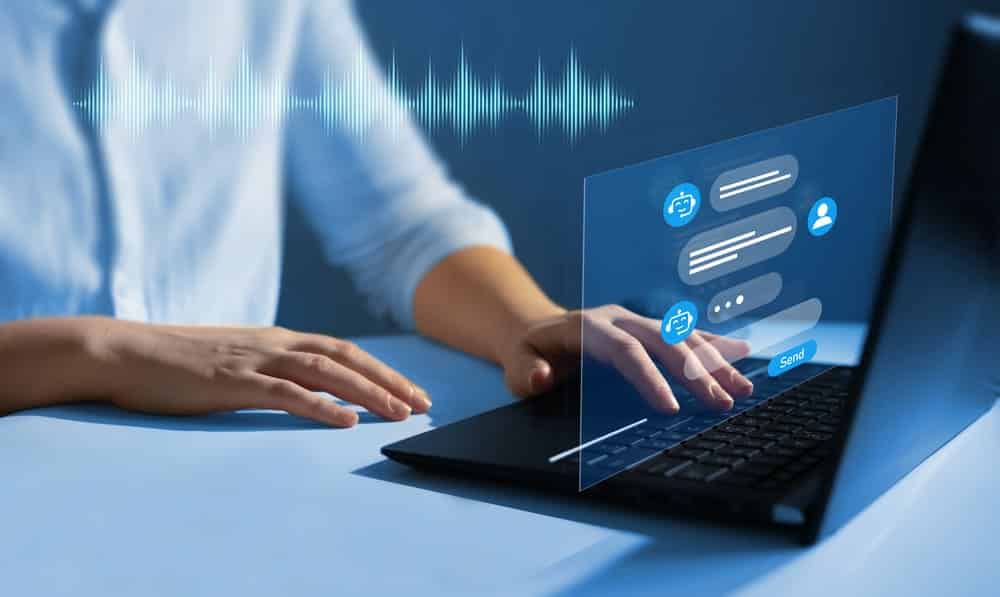
Editor’s note: This article draws on insights from a CIO Dive virtual event panel. Register here to watch a replay of the full event, “AI and Managing the Data Underbelly.”
CIOs have prioritized data management and modernization for several years — if not longer. But like with the rest of the tech stack, CIOs are reexamining data pipelines as enterprises shift to generative AI adoption.
“Getting all this data to the right place at the right moment – it’s not an easy task,” Alon Amit, VP of product, analytics, AI and data at Intuit, said during a CIO Dive panel. Generative AI has only intensified the challenge.
CIOs navigating adoption initiatives are leaning on a variety of data sources, emphasizing the deliberate curation of training sets and operating with elevated urgency.
“One of the biggest pieces that have changed for us is knowing that it’s okay to run a proof of concept and have it not work exactly how we think it should,” said Shawna Cartwright, business information officer and SVP of enterprise technology at Cushman & Wakefield.
When it comes to generative AI, failed experiments aren’t strictly chalked up as bad for the business. Failing fast, a tenet of tech startup culture, can work for enterprises, too.
Cushman & Wakefield’s goal is to embed AI across the commercial real estate transaction lifecycle. The company laid out an AI initiative in November, aiming to improve productivity and assist employees with day-to-day tasks.
“Everybody in the company, from the top down, has to truly understand that failing fast is something we all want to do because if we’re not failing that means we’re not learning and we’re not growing,” Cartwright said.
Tech chiefs rethink data sources
As organizations take stock of their data estates, extracting value from previously overlooked unstructured data has become an attractive proposition.
“Other than scale and speed, it’s also the variety of data that we now care about and the relative importance of those datasets has changed, sometimes dramatically,” Amit said.
Intuit turned to an untapped data source — the company’s blog posts — to train models to speak more to the fintech company’s domain.
Cushman & Wakefield has a similar end goal. The commercial real estate firm is exploring the use of informal, written documentation to give generative AI tools deeper institutional knowledge.
“Those are things that we’re testing out at the moment,” Cartwright said. “We don’t have full answers to how we’re going to do some of it.”
It’s a quest that many organizations have embarked on since experimenting with generative AI. In the banking industry, for example, organizations are looking to expedite contracts and audits with generative AI tools, according to Michael Abbott, senior managing director and global banking lead at Accenture.
Banks often have enormous legacy systems, and the highly regulated nature of the business pushes leaders toward acquiring data and AI solutions from niche vendors, Abbott said. When applicable, businesses are beginning to cost-optimize tools.
Most banks have likely taken advantage of opportunities with structured data, but generative AI has opened a new door, Abbott said.
“It’s really all the unstructured problems out there right now that are being unlocked for the first time,” Abbott said. “This is perhaps one of the most transformative things we’re going to see in the next five to 10 years.”
This article was written by Lindsey Wilkinson from CIO Dive and was legally licensed through the DiveMarketplace by Industry Dive. Please direct all licensing questions to legal@industrydive.com.
Photo Credit: Galeanu Mihai, Getty Images
Best of the Month. Straight to Your Inbox!
Dive into the best content with our monthly Roundup Newsletter!
Each month, we handpick the top stories, insights, and updates to keep you in the know.