How Morgan Stanley Streamlined Content Creation and Access With Machine Learning
Create content once and use machine learning to customize it to varied access channels.
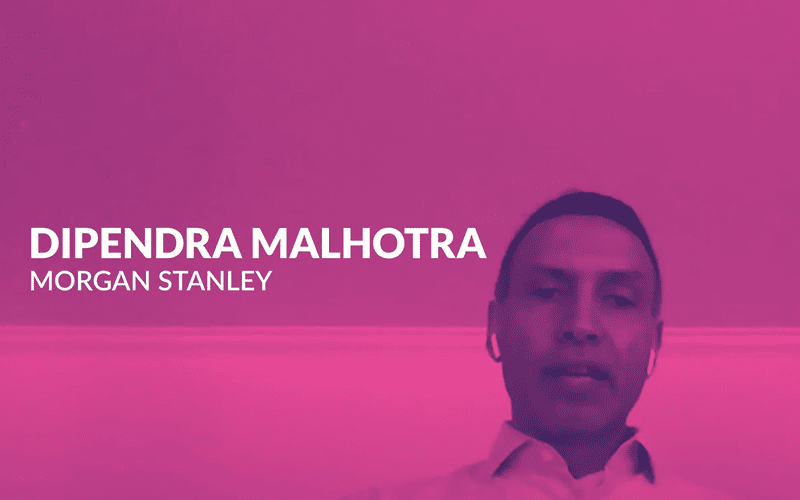
A company’s most valuable resource lies in its employees. That’s what Morgan Stanley’s Head of Analytics, Intelligence, and Data Technologies, Dipendra Malhotra, recognized and shared in his talk “Optimizing Content Once for Search, Chatbot and Voice” at 2020’s Activate, the Search and AI conference.
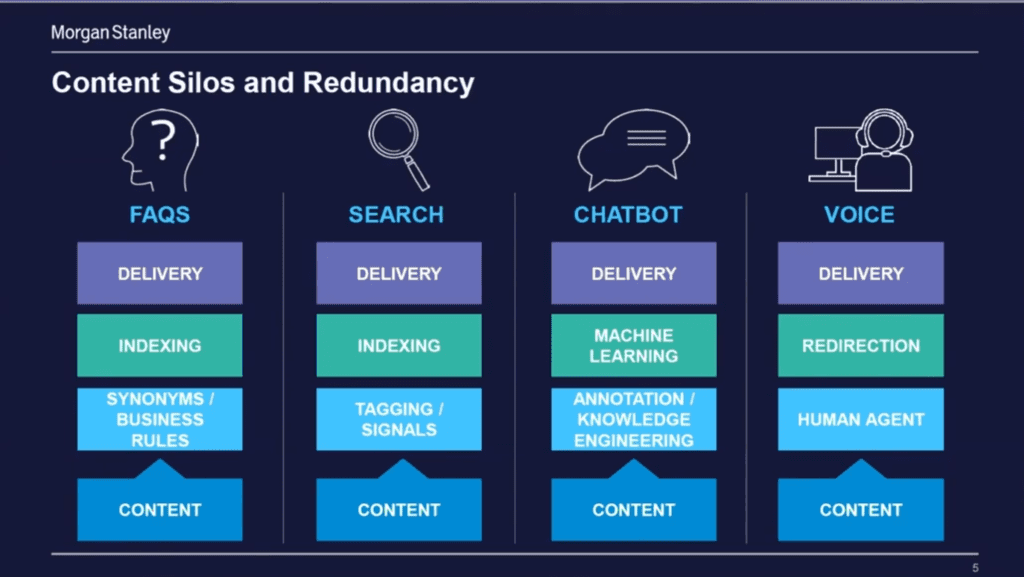
Morgan Stanley’s before state.
With employee time at a premium, reducing repetitive work can mean large cost savings to an organization. In the case of content creation, organizations save valuable time reusing documentation produced by the firm’s subject matter experts (SMEs). After content is created for the knowledgebase, the team uses machine learning to customize that content to the channel and the person searching for information.
“It became very important for us to really focus on the content once, because that’s where the human capital is utilized,” Malhotra explained. “Whether that content is rendered on search, on chatbot, voice, or FAQs, it really doesn’t matter at that point because all those channels can be automated.”
Many Points of Entry, Lots of Information
At Morgan Stanley, complexity gets layered into accessing its knowledge corpus with the breadth of the firm’s offerings (financial planning, advisory services, transactional support, cash management and lending) and the diversity of its users. Morgan Stanley has 15,000 financial advisors managing $2.6 trillion of assets, serving 3 million clients, plus branch support staff and service agents – all of whom query the knowledgebase in a slightly different way, with a slightly different purpose. Also, the vehicle used to arrive at the solution can come in many forms: an FAQ document, chatbot, search, or voice.
Traditionally, because of the various formats required for responses, content that serves identical query intent gets created many times over. If an SME writes an FAQ, even adding synonyms and business rules to optimize it, because a chatbot application requires content in a more conversational format, the FAQ content must be recreated for the chatbot. And so on recreating, retagging, and customizing content for each vehicle. The amount of human capital required is high.
Create Content Once, Use ML to Customize Responses
Malhotra’s team built a freeway to the solutions available in Morgan Stanley’s knowledgebase by using a tested process and the indexing and machine learning capabilities available in a chatbot platform and Lucidworks Fusion.
Starting with an ontology to house the knowledgebase, now when an SME creates content, they also add synonyms, tags, and hierarchy rules. All of that content is loaded into Fusion for indexing, and machine learning takes over, rendering the content appropriate to the channel on which it was asked.
Malhotra used the example of a customer looking for information with the intent of opening an account. The customer may go to Morgan Stanley’s search platform and type in “I want to open an account” or “how to get an account.” The first thing that has to happen is that the computer needs to interpret what exactly is being asked.
“Open” is tagged as the intent and “account” is tagged as an entity. Is the “I” in the query a financial advisor, a client, or a service agent? This provides the machine with the context it needs to understand how similar users have behaved, or if its an authenticated user, how this exact user has behaved in the past. Does the channel this customer is asking on have a specific response already customized for this query?
Fusion will render relevant search results for this particular “I”, that are tagged with intent: “open”, entity: “account”, customized to the channel on which the user is asking.
To better serve the user, more details can be extracted from their query to understand their intent. Continuing the “open an account” example, many types of accounts exist. The chatbot can ask “what kind of account do you want to open?” If the customer responds “IRA” then query results will explain how to open an IRA account specifically.
Reducing Redundancy, Improving Consistency
Machine learning in Fusion has ultimately allowed Malhotra’s team to reduce the repetitive work required of its SMEs, saving the organization superfluous costs associated with mismanaged employee time, and better serving its customers with consistent information customized to each query channel.
![]() |
“The most expensive item in this whole chart is the content field at the bottom, because that’s where you have your SMEs creating that content, thinking about the questions, thinking about the answers, thinking about the search, thinking about what needs to be rendered on the search. Everything else can be done very quickly and through the machine.” |
Interested in hearing more about how Lucidworks helps organizations across the Global 2000 connect people with insights? Check out this ebook on how Lucidworks empowers financial advisors: saving them time by putting recommended research at their fingertips and helping them build a loyal clientele.
Best of the Month. Straight to Your Inbox!
Dive into the best content with our monthly Roundup Newsletter!
Each month, we handpick the top stories, insights, and updates to keep you in the know.