Video

Video
When AI Goes Wrong: Real-World Fails and How to Prevent Them
Don’t let your AI chatbot sell a $50,000 Tahoe for $1! This...
Read More
Video
Lucidworks Core Packages: Industry-Optimized AI Search & Personalization Solutions
Discover our comprehensive Core Packages that combine Analytics Studio, Commerce Studio, and...
Read More
Video
Product Demo: Neural Hybrid Search in Action (New Pig)
Watch as our VP of Solution Engineering, Brian, demonstrates how New Pig...
Read More
Video
Analytics Studio: Transform Your E-Commerce Data into Actionable Insights
Discover how Analytics Studio empowers teams to make data-driven decisions without needing...
Read More
Video
Commerce Studio: Personalize Your E-Commerce Experience in Real-Time
Explore how Commerce Studio delivers tailored shopping experiences that drive conversions and...
Read More
Video
The Incredible Limitations of Build-It-Yourself Search
Why DIY Search is Slowing Your Team Down
Read More
Video
5 Takeaways From the World’s Largest Generative AI Study – KMWorld Podcast
Maximize Business Value with Generative AI: 5 Takeaways From the World’s Largest...
Read More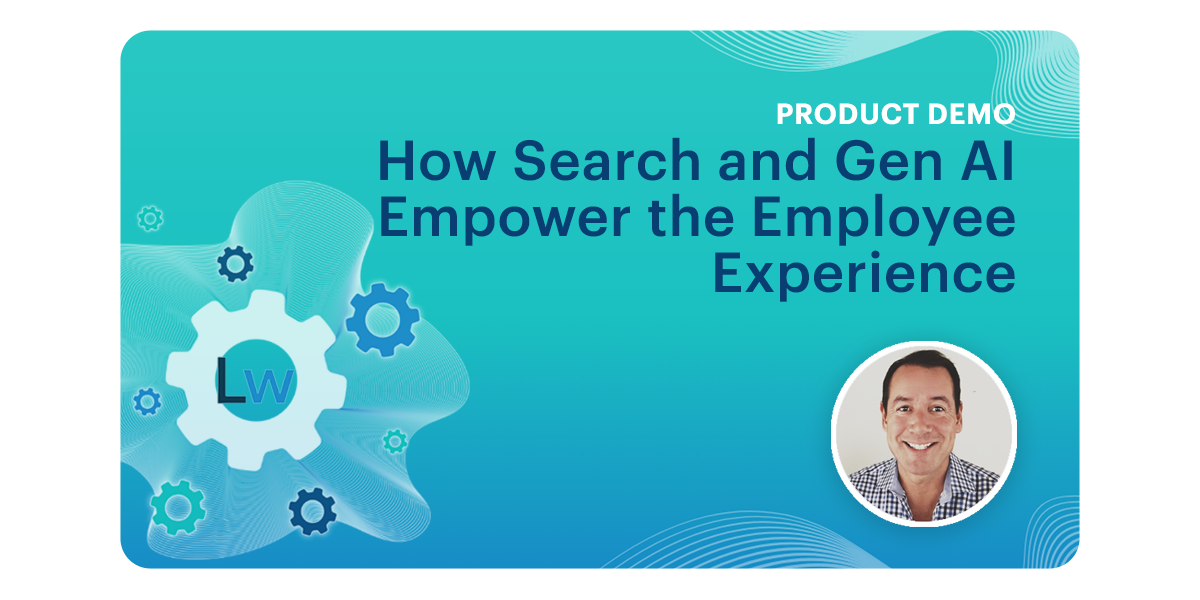
Video
Product Demo: How Search and Gen AI Empower the Employee Experience
Enhance knowledge management with AI! In this demo, VP of Sales Engineering...
Read More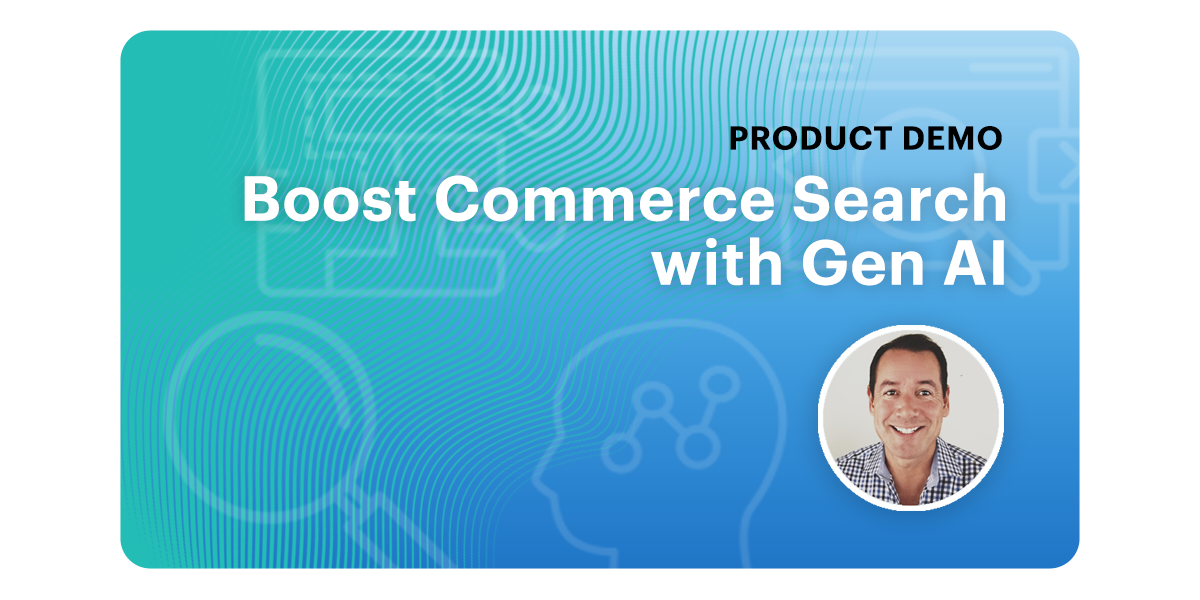
Video
Product Demo: Boost Commerce Search with Gen AI
Discover how generative AI transforms your commerce search experience. In this demo,...
Read More