7.39%
support case deflection rate
311%
increase in self-solve rate
2.2
average click depth
How an enterprise technology company increased its self-solve rate by 311% and deflected thousands of support cases through intelligent search.
“We were able to determine that Lucidworks was the best way that we could engage our customers by delivering information faster.”
— John Paul Sherman, Enterprise Search and Findability Manager, Red Hat
Customers prefer to fix their own problems
When enterprise technology company Red Hat A/B tested their customer support homepage, offering a self-service option to some visitors and not to others, the results were clear.
“There was an increase in traffic that confirmed customers are really motivated to self-solve, and we saw a 7% decrease in support case creation for customers who were given the self-solve homepage.” — Manikandan Sivanesan, Principal Software Engineer, Search, Red Hat
This insight led Red Hat—a provider of open-source software products and solutions to a global customer base, including over 80% of the Fortune 1000—to reimagine how they deliver customer support through their award-winning portal.
“A critical part of our customer’s experience is to find information when things break. Search is really kind of the front line for where people look. If they’re able to find the right content, then they’re generally happier. They’re more likely to renew their subscription.” — John Paul Sherman, Enterprise Search and Findability Manager, Red Hat
The triple win for self-service
Red Hat recognized that promoting self-service creates three distinct benefits:
- Customers win by getting the answers they want quickly, similar to the experience they expect from an ATM or self-checkout
- Support engineers win by focusing on complex outlier requests that require engineering acumen rather than handling routine issues
- The business wins through reduced operational costs, as self-service transactions cost pennies compared to $13+ for a typical B2B live service interaction
The challenge was making self-service truly effective, especially for a company with over two dozen products and hundreds of thousands of knowledge articles, product installers, and technical resources.
Understanding customer intent
To create an intuitive self-service experience, Red Hat needed to understand the intent behind each customer’s visit to the support portal. Are they trying to:
- Solve a problem using resources in the knowledge base?
- Download product software or installers?
- Troubleshoot a specific error message?
- Open a new support case?
By leveraging Lucidworks’ machine learning capabilities, Red Hat built a system that detects the intent behind a user’s search query and directs them to the most appropriate interface. If the model infers that a user is searching for an error code, for example, they’re sent to a dedicated troubleshooting tool designed to make the experience intuitive.
“What we found with Lucidworks was the ease of configuring the data so that machine learning works. That was incredibly important to us, not just saying that there’s machine learning and artificial intelligence, but the level of support and the level of data that we can provide the machines to consume to make our search better.” — John Paul Sherman, Enterprise Search and Findability Manager, Red Hat
Learning from multiple feedback loops
Red Hat’s approach to relevancy uses two powerful feedback loops:
Customer behavior signals
The system captures comprehensive clickstream data: which articles customers click, what pages they visit, how long they stay on each page, and more. This data is aggregated using Lucidworks Signals Beacon to understand which content is truly helpful.
“If a user searches, clicks on a piece of content, scrolls all the way down, but only lasts on the page for two seconds, we now know that that may not be the best result for that particular query. But if they stay for four minutes and they scroll all the way down, that sends a signal that this may be a good result.” — John Paul Sherman, Enterprise Search and Findability Manager, Red Hat
Support engineer insights
When a customer opens a support case, the support engineer records diagnostic data and attaches any knowledge base documents used to solve the case. This creates a valuable association between problems and solutions that benefits future users.
Queries are run against this support case collection, and when a similar issue is identified, the solution linked to the previously solved case is surfaced. The system applies a popularity measure that boosts documents based on how many cases they’ve resolved, with a time decay function that prioritizes recent solutions.
Bridging language gaps with AI
Red Hat’s open-source products create unique search challenges. Customers often search for upstream components rather than Red Hat’s product names, so traditional keyword matching isn’t sufficient.
By coupling Lucidworks with Word2Vec, a natural language processing technique, Red Hat can identify word relationships and solve language mismatches. For example, when customers search for “Kubernetes” when they’re actually looking for information about Red Hat’s OpenShift, or “Karaf” when seeking help with Fuse, the system can make these connections.
Measurable results that matter
The improvements to Red Hat’s self-service portal have delivered impressive results:
- Over two months in 2020, the support case deflection rate reached 7.39%, with more than 2,700 support cases deflected
- Compared to October 2018, when the deflection rate was just 1.8%, this represents a 311% increase in the self-solve rate
- Customers find what they need efficiently, with click depth averaging just 2.2 clicks and click-through rates averaging 58.4%
“When faced with any questions about product usage or errors, customers need the option to turn to knowledge-based platforms. If these provide accurate and relevant content, customers will use them willingly rather than reaching out to a service representative. It puts them in the driver’s seat and gives them control.” — Manikandan Sivanesan, Principal Software Engineer, Search, Red Hat
The road ahead
For Red Hat, which has been recognized by The Association of Support Professionals as having one of “The Ten Best Web Support Sites” for nine consecutive years, the journey toward even better self-service continues.
“There are a lot of plans, a lot of very tactical plans that we want to use in conjunction with the Lucidworks Platform to make our search results better,” Sherman shares. “These are projects I’m incredibly excited about.”
The company’s success demonstrates that with the right understanding of customer needs and the right technology to deliver relevant information, self-service isn’t just a cost-saving measure—it’s a competitive advantage that drives customer satisfaction and loyalty in the subscription-based software business.
By implementing intelligent search that understands customer intent, Red Hat increased its self-solve rate by 311%, achieving a 7.39% support case deflection rate and helping customers find solutions in just 2.2 clicks on average—proving that in technical support, empowering customers to help themselves creates better experiences and business outcomes.
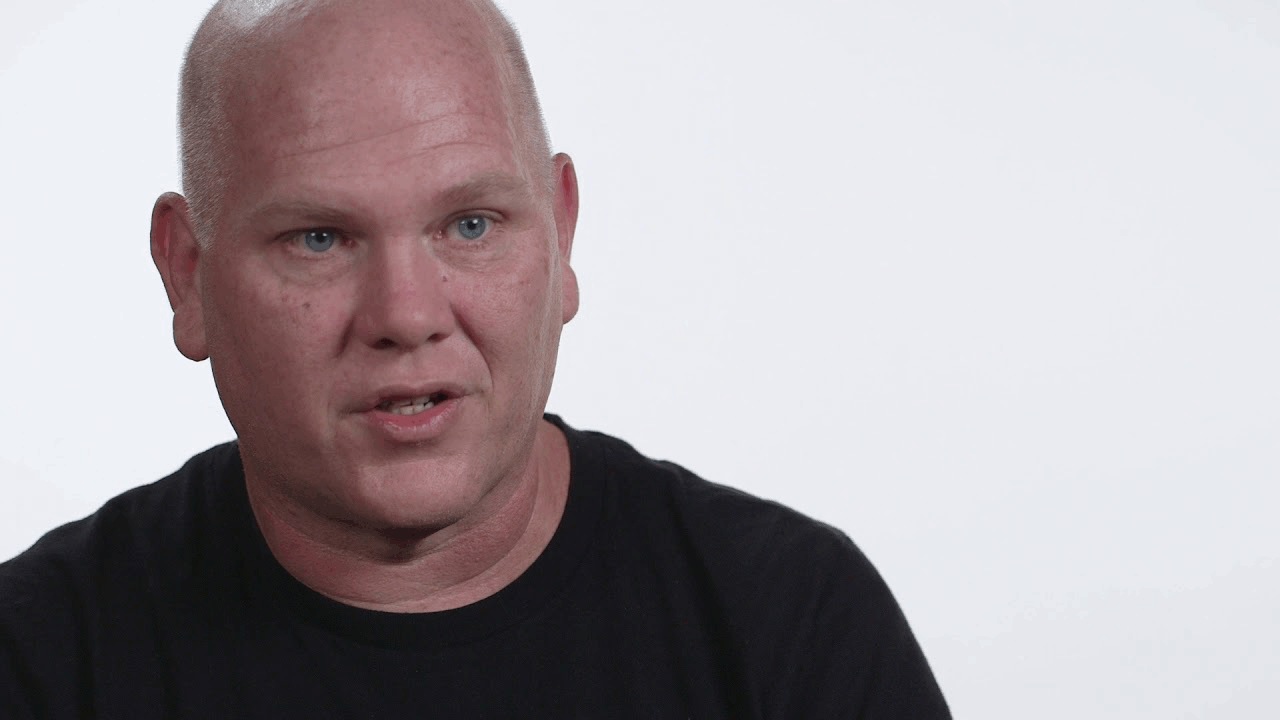