Pharma Is the New Google
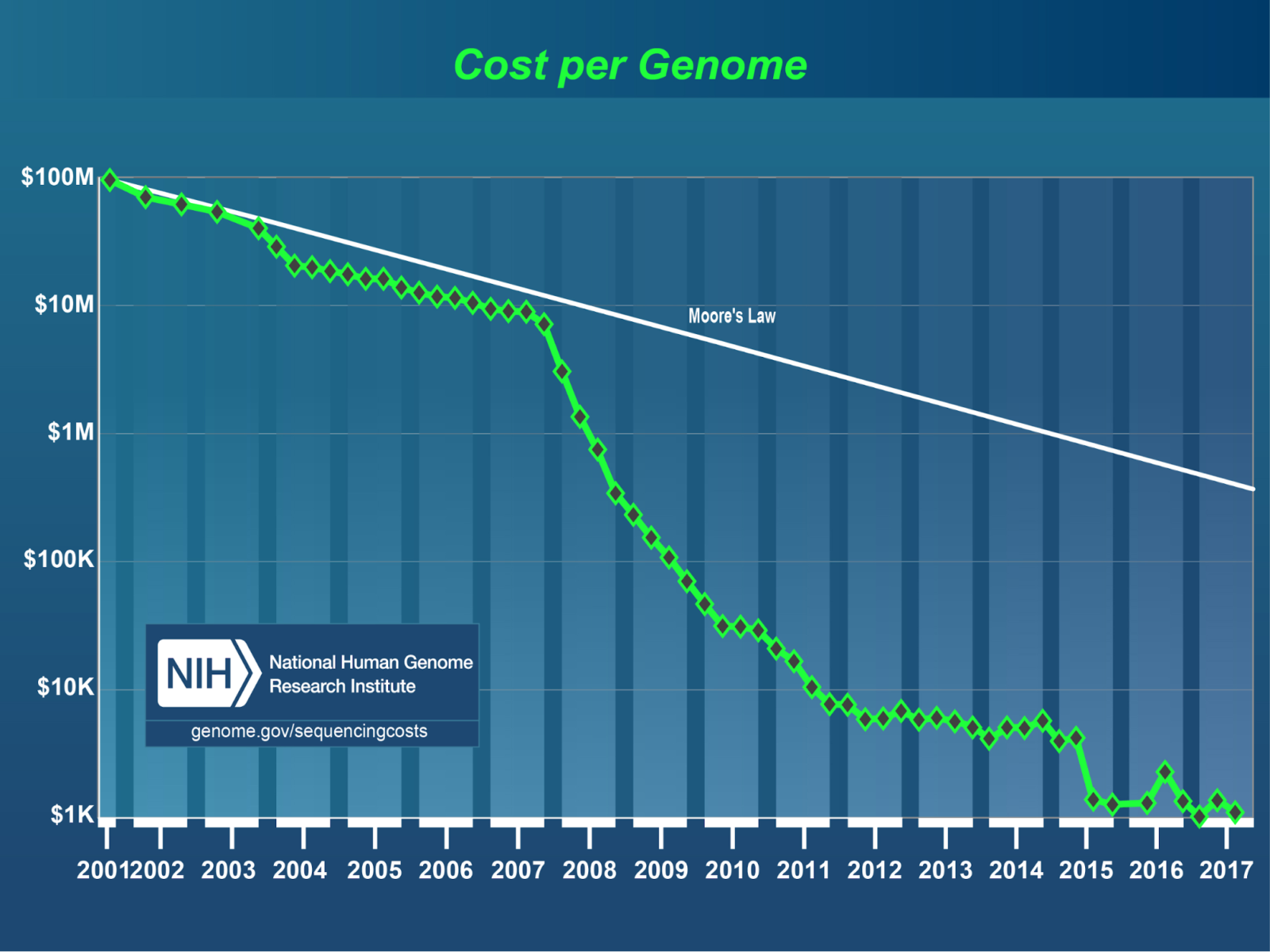
The traditional method of drug discovery in the pharmaceutical industry is failing. There are fewer drugs coming out on the market. Those drugs are developed at a much greater cost than ever before and those costs are increasing. There are only so many mechanisms of action that are common among everyone and treat a major disorder with an acceptable set of side effects. So the industry has been looking less toward “blockbuster” drugs and more toward treatments for increasingly rare diseases and disorders. Those rare disorder medications command a premium instead and require fewer clinical test subjects to develop. However, payers may not be willing to pay those premiums.
The Personalized Medicine of the Future
There is, however, considerable cause for hope in the industry. New technologies are making a more personalized form of medicine possible. The cost of sequencing a person’s DNA now costs less than an MRI (http://time.com/money/2995166/why-does-mri-cost-so-much/). This has helped give birth to a new field of medicine called pharmacogenomics.
Pharmacogenomics may soon allow the creation of drugs, drug therapies, and biosynthetics that target individuals or classes of people with specific genetic variations. A subset of this, oncogenomics, aims at targeting cancers that specific individuals are genetically more susceptible to, while navigating the toxicity and lethality of synthetic drugs for each person. This new field of personalized, gene-centered medicine may allow for more effective treatments and reinvigorate the pharmacological pipeline from a business standpoint.
However, personalized pharmacogenomics requires that the current state of the pharmaceutical industry change. First of all, it requires new business models. It won’t be enough to simply throw a drug on the market and market it. Instead, the pharmaceutical company will need to be more closely involved in treatment and will need to continuously gather data about treatment and treatment effectiveness.
Medicine is Data
In essence, if a pharma company isn’t already a data company on par with Google or Facebook, they must become so now. All of the R&D, treatment, and marketing will create a ton of data.
Rather than a unidirectional process of research, clinical trials, then treatment in the field, the development process will become continuous and more patient-centric. As patients are treated and data is gathered about efficacy and negative effects, new approaches and new treatments will be adapted — at the genomic level — generating ever more data along the way. Development of these treatments will start in the computer instead of in the laboratory. This type of research and development has actually been happening for a while now, but is about to become pervasive and standard.
Managing and matching that data to its purpose and then evolving that data over time will require a completely new approach, different from the traditional big data warehouse or big data lake. Those may still end up being a part of the process, but they are not sufficient. This new approach means that we’re forever searching for data, in real time, over different sorts of datasets, and in ever evolving forms.
AI in Pharma
When we think about finding the right compound or sequence for an individual genome, it is essentially a matching problem, over a large amount of data. This kind of problem is exactly what AI approaches, like machine learning, were designed to do. What a researcher does in terms of applying a process, learning about the results, and using that experience to try something new, is exactly what deep learning is designed to do.
The new research lab starts inside of a computer and extends to the field. The new most valuable researcher on your team might be an algorithm. These algorithms and AI models may end up transforming the pharmaceutical industry in the same ways that they transformed finance and high frequency trading.
Your Biological Data Are Your Signals
Ecommerce companies like Amazon and search/data companies like Google have used behavioral signals, like what a user clicked on, scrolled through, or bought, to profile user behavior and influence purchases.
Medicine has the same kind of problem. There are biological signals (responses to medication, effects, side effects) and behavioral signals (did the user take the medicine, sleep, run, walk) to track, record, and compare. The optimum course of treatment may be a combination of biologics, drugs, and lifestyle changes necessary to achieve optimal health, cure of a disease, or at least alleviate the symptoms of disease. The same kinds of algorithms that Google and Amazon use to influence behavior are the same kind of algorithms that a treatment may require.
How Can This Work?
A key challenge is managing this data and developing, applying, and refining the algorithms and models. Unfortunately, existing data systems either require heavily structured static approaches to data or are just big fat filesystems that require each user to learn everything about each data structure before making use of it. Moreover, both systems require laborious external data cataloging and curation systems.
The data system of the future for pharma requires handling unstructured text or numeric data, semi-structured changeable schematic data, as well as structured data. The data needs to be discoverable and self-documentable. The data system needs to be able to scale as ever-increasing amounts of data are gathered and used in real-time.
Data transformations need to be addressible in stages and allow multiple evolving algorithms and models to be applied. Data also needs to be distributable, exportable, shippable, and usable on a global basis. AI algorithms and models have to be managed, maintained, applied, and tested. All of this requires a mature but future-looking platform.
Learn More
Best of the Month. Straight to Your Inbox!
Dive into the best content with our monthly Roundup Newsletter!
Each month, we handpick the top stories, insights, and updates to keep you in the know.