Play Video
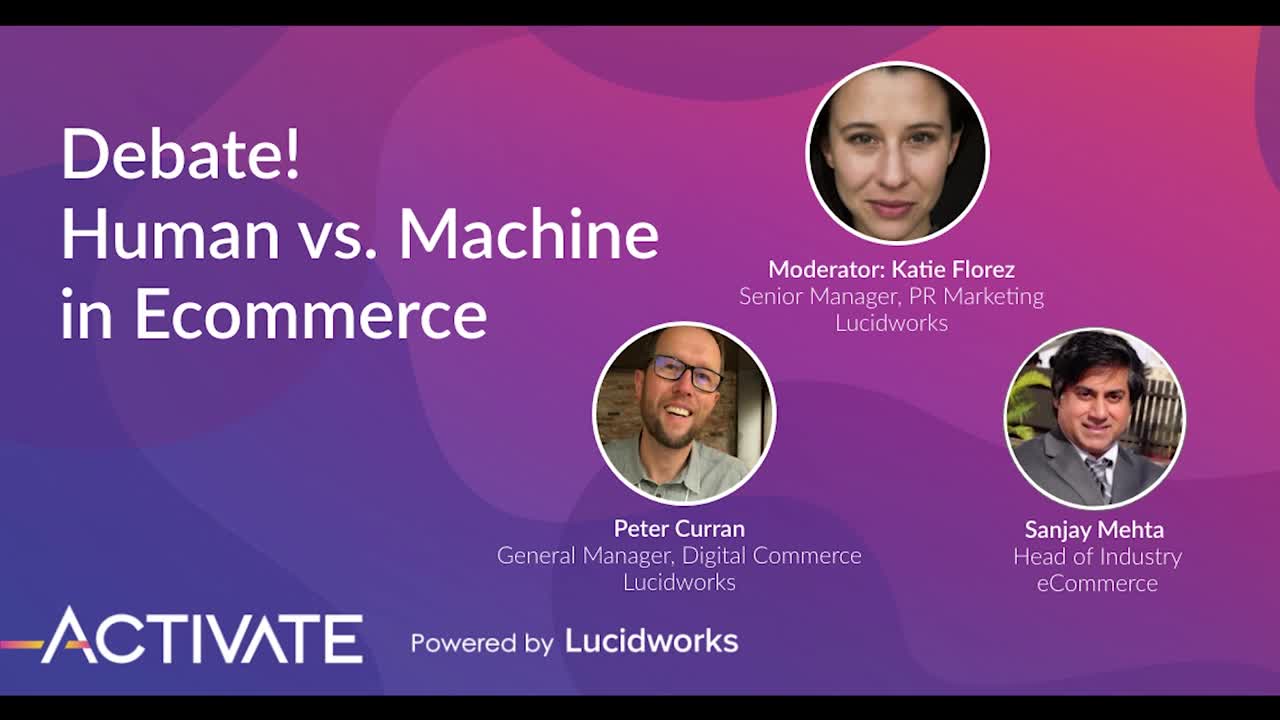
Watch a spirited debate about the future of ecommerce experiences. A moderator will ask a series of questions that explore the limits of machine learning in ecommerce and the impact on both customer and operational roles such as marketing, merchandising, and customer service.
Intended Audience
Ecommerce and customer experience leaders
Attendee Takeaway
A better understanding of the major pros and cons of adopting and operationalizing machine learning in an ecommerce context.
Moderator
Katie Florez, Senior Manager, PR Marketing, Lucidworks
Speakers
Peter Curran, General Manager, Digital Commerce, Lucidworks
Sanjay Mehta, Head of Industry, eCommerce
[Katie Florez]
Welcome everyone. We’re doing things a little differently in today’s session at Activate. My name is Katie Florez, and I am the Senior Manager of PR and Marketing here at Lucidworks. I’ll be your moderator today, and I’m really thrilled to have you all here for our debate. The topic of discussion is, should e-commerce operations prioritize investments in machine learning over investments in human curation? Our two panelists joining our battle royal today are Peter Curran, the General Manager of Digital Commerce here at Lucidworks, and Sanjay Mehta, Head of Industry E-Commerce, also here at Lucidworks.
Sanjay will be representing the need to prioritize investments in machine learning, and Peter will be representing the side of human curation. I’m going to lay out a few ground rules here before we start. After we ask each question, I’ll give each debater between 30 seconds to two minutes to respond. As much as you may want to, Sanjay and Peter, you are not allowed to talk over your debater, or use any unethical distraction techniques, not limited to changing your Zoom background to outer space, or putting a cute puppy or kitten in front of the camera. Okay, fair? Ready?
[Peter Curran]
Dang it.
[Katie Florez]
Awesome. Let’s do this. Sanjay, I’m going to start with you. Why should we embrace machine learning? I’ll give you two minutes to respond.
[Sanjay Mehta]
Okay. I think I’ll break it down to four main areas. One is automation. And you know, the ability to do things in a more scalable fashion. And then another topic I think that’s important is reaction time, which is the ability to make decisions instantaneously on mass amounts of data. And then finally, precision would be a big one. Getting things based on underlying data versus using a hunch.
And I think, really where we’ve come along, even really, with birth of e-commerce, particularly, but all the digital touchpoints we have now is an enormous amount of data. And it’s difficult for the human mind, in any way, to process and make decisions with all that data at scale. And when we look at our minds not really being purpose-built for that task, but more dealing with smaller groups or individuals, I think that’s kind of where machine learning has come along and been purpose-built for that task.
And so, when we think more about engagement of a user in real time, and looking at millions of users, and understanding what they’re doing, and reacting, I think that is obviously something that’s almost impossible for a human decision maker to do.
[Katie Florez]
Right. And that’s definitely an interesting argument around scalability and automation. Peter, I’ll give you two minutes to respond to Sanjay.
[Peter Curran]
Yeah, thanks. First, I’ll say that, as somebody who works for a company that sells machine learning technology, it’s difficult for me to take the side of the humans, but I’m gonna do my best. And I fully believe the arguments I’m gonna make to you today.
The first is that machine learning is a secondary or tertiary consideration at best, for most retailers and B2B e-commerce sites, at the enterprise level. There are usually many more, much more important priorities around things like structuring product data correctly, getting your categories organized the right way, making sure that your site is tagged properly. How many times have you looked at your metrics, and put an asterisk next to them, and saying, oh yeah, but because it’s not tagged right, that conversion rate is wrong, or something like that? Virtually everybody in the audience has experienced that kind of apology for your metrics. Things like, your basic keyword search, is it optimized for your top 200, 500 terms? These kinds of things, they’re related to machine learning, but I think they come before machine learning. That’s the place where we should focus our investment.
Machine learning is subject, as technology has always been, to the garbage in, garbage out problem. And so my argument would be, we should prioritize human investments, to sort of set the stage for quality machine learning.
The second argument I would make is that the downside risks are significant. So I kind of look at machine learning as sort of, it’s not a perfect equivalency, but sort of like the nuclear technology in the realm of computer science of our age. And even now, what, 80 years on from creating nuclear technology, we still don’t control it very well. Just look at the Fukushima disaster from 2011. And it’s clear that we don’t control machine learning very well.
Just in the last week, Facebook is gonna be dragged in front of Congress yet again, to explain why it knew that its algorithms exploited children, and caused them to get addicted and overly engaged in their applications, yet continued to make investments to drive children towards more use of their application. So, I think there are moral and ethical considerations that we haven’t thought about. So all of this automation reaction time and precision stuff that Sanjay is talking about is all well and good, we just don’t control over this technology yet.
[Katie Florez]
Great. So the importance of human oversight over things that are simple, maybe not simple, but that are smaller, metrics and tagging, but also the larger moral and ethical considerations are reasons for prioritizing human investment. Sanjay, I’ll give you 30 seconds just to respond to Peter since we had you start us off.
[Sanjay Mehta]
Sure. If I break down sort of the argument of poor data first, yes, I do believe there’s a lot already in place, in most digital platforms, in the form of analytic packages, and tag management, that folks have already been using, even to measure their business that now are available to AI. So I think part of that problem, and a lot of folks are investing in master data management, product information management tools, really to kind of manage and control the structure of their data, to make it more usable.
So I think a lot of that, from what we observe, has been there. And one argument I’ll grant is, the poor experiences, a lot of it is using the wrong tool for the wrong job, right? I think we all heard using a hammer to put in a screw. A lot of it is, yes, was configured improperly, or, that’s kinda where some of the human impact comes in, is choosing the right models, and also incorporating what we call explainable AI, to let you know what the machine’s doing and why, I think is something more folks should start using now.
[Katie Florez]
Great, thank you. Peter, before we move on to the next question, is there any thing you’d like to respond to Sanjay with? I’ll give you about a minute here.
[Peter Curran]
Yeah, I mean, Sanjay and I park our cars in the same garage on that one. The business user needs, not the data scientist, not the team of engineers, the business user needs to be informed about what the machine learning technology is doing. And we’re just not really there yet. I mean, Lucidworks has a great, deep learning based product discovery technology that allows us to find products that are outside of the retailer’s, for example, vocabulary.
It’s really difficult to explain how that works so that a business user can take control of it. So, I think as people working in the industry, we can develop this really incredible technology, but we need to go a lot further, in terms of making it so that the business user, who unlike a machine, has a true understanding of the product and of the customer, so that they can understand what that technology is really doing.
[Katie Florez]
So I’m hearing you say that there’s some importance and challenges in having humans and technology work together to create more value all around, and that sounds incredible and impactful in theory, but I’ll pass this to Sanjay, you know, what are some real life examples of where human curation and machine learning can work together?
[Sanjay Mehta]
Yeah, I think certain tasks that are very monotonous or redundant, that is a really great opportunity or low-hanging fruit to be automated, or owned by machine learning. And if we think about e-commerce, a lot of it is, the browse and navigation, which, again, is very difficult, even for a human to individualize, or make more relevant for each individual user, but it’s very easy for the AI to take over.
But with that said, there always might be a use case that there’s a strategy in place by the business that they want to influence what users see. We all know that, we’re pushing our marketing, certain items, we’re promoting certain content. And therefore, I think there’s always that room to override what the users are seeing, or their exposure to the machine learning. And I think what we hear out there is really more using machine learning to manage more of the rule, and the humans kinda manage on exception. Or the 80/20 rule you might hear a lot, right? Is use the machine to do majority of the work that you know is repetitive, for example.
[Katie Florez]
And Peter, specifically in regards to business users, do you think that they’re prepared to do this kind of work, with bringing together human curation and machine learning?
[Peter Curran]
Sorta. I think there’s dignity in all work, first of all. So we need to be a little bit careful passing judgment about whether this work is appropriate for a person to do, or that work is inappropriate for a person to do.
You look at like, I think it was last year, Walmart hit the news because they had canceled a contract with a robotics company, where they had these robots in their stores at night, checking stock levels on shelves, they canceled the contract because they found that humans could do that better. And there are people out there who want, and desperately need those kinds of jobs. So, I would be a little bit careful with that.
I would also say that when you allow AI to take over, sometimes AI steers in a direction that you don’t want. So if you are a brand, like, particularly, think about it, like a fashion brand, you don’t necessarily want to chase conversion at all costs. There are certain products that you want to sell because they’re higher margined, in season, full-price products. If the machine learning chases, for example, a little bit too much of a focus on conversion, then you’re going to be selling a lot of discounted products, or leading people to products where the sizes are broken, or something like that.
So, I think that there are some of these kinds of controls and opportunities, but in a lot of cases, some of the finer points are missing.
[Katie Florez]
Sanjay, I’ll give you 30 seconds to respond to that, if you have anything you’d like to add.
[Sanjay Mehta]
Yeah, I think it goes more down to, yeah, doing an AI assisted model in some of those cases, right? Which is the AI kinda shows you, which is something we all also expose through our merchandising tools, for example, where the AI sort of shows you what it thinks, and then you as a business user can decide if you wanna go along with that, or override it.
So again, and then there’s a lot of those edge cases, or long tail, where you can’t get to all those different scenarios your users may go through. And for that, if you wanna call it a fall back, you have the AI, they’re at least doing something for you. I mean, the other piece we’re hearing is, more recently too, is that the shortage of resources, for example, a lot of people, whether it’s in fulfillment or operations, there’s a lack, you have large organizations like Amazon sucking up all this resource and talent, where they don’t even have the staff to do certain things to improve their results. So they have a fall back here, with the AI, in my opinion.
[Katie Florez]
Thank you. I wanna shift gears here a little bit, and Sanjay, I’m actually gonna pass this question right back to you. So some people argue that to have this true, one in a million, you get me type of customer experience, you need the genius and intelligence of a human. But for top retailers who have hundreds of thousands, even millions of customers, can you really provide that level of true one-on-one personalization using machine learning?
[Sanjay Mehta]
Yeah, I definitely believe so, because it’s impossible for, even if you had 10,000 business users to create those one-on-one experiences, just because you’re always gonna have more users than business users. I mean, that’s a simple one. But I think going back to the one-to-one, first of all, with the age of privacy, and being able to get third party data, for example, that’s pretty much gone away, we lean on observing behaviors.
And that’s something the machine can do really great, is observe behaviors, and similarities in behaviors to create an experience that works for each user. And I think it goes down to what we call more of a predictive approach, which is really more one-on-one, based on listening to the user, versus a prescriptive approach of telling the user what they need to see. And I think that it’s important, that you might have a small mix, where you do want to own your brand, you wanna control the experience, but then again, users wanna be heard. They want that site to listen to them, and react to their intent. And so that’s my-
[Katie Florez]
Great. Great, thank you. And Peter, I’ll give you a minute to respond to that, if you’d like.
[Peter Curran]
You’d better have full control over it, right? The downside risk is serious. I love that Tweet from a couple of years ago by Jack Reynor where he says, dear Amazon, I bought a toilet seat because I needed one. Necessity, not desire. I do not collect them, I’m not a toilet seat addict, no matter how temptingly you email me, I’m not going to think, oh, go on then, just one more toilet seat, I’ll indulge myself. Right?
That’s the downside risk, that the thing starts to try to know you in a way that’s just obviously wrong and stupid. And so, if you’re going to go down the road of a more one-to-one, hyper personalization type approach, you need to be able to interrogate the signals that are driving the decisions that the AI is making. You need to make sure that business users can inhabit the personality of a customer who’s going to receive an offer of some kind. You need features like this that allow the business user to fully understand the scope and ramification of the technology that they’re unleashing on their precious customer.
[Katie Florez]
And thinking about that downside risk, I know you were talking about it in the context of machine learning, but I also wanna flip it and throw it back to biases as humans, how we curate experiences for our shoppers. If I go to REI, and I type in backpack, and I actually want some different kind of bag than what is popping up on the site. How do I, as a retailer, address those maybe unconscious biases that I have as a merchandiser, about how I’m curating that experience? ‘Cause that to me, would be the other downside of the risk, as investing more heavily in human curation.
[Peter Curran]
I think you test. So you do automated regression testing on the curation decisions that you make, to understand, this is the thing I love about e-commerce, is that it’s a market. And just like in a normal market interaction, you get feedback from the customer. If you build a rule, and then the conversion rate starts to dip around backpacks, maybe that’s an insight that, it could be that it’s just not the season to buy backpacks anymore. All the ones that are good are sold through, and it’s only the crummy ones that are left. And it could also, on the other hand, be that you’ve been overly specific about your rule. So I kinda think that those feedback mechanisms are just inherent in the operations and diligent monitoring of an e-commerce site. You know, again, it’s a little hard for me to argue this perspective, but that’s the way I would flip it back to you.
[Katie Florez]
Absolutely. Sanjay, I’ll give you a quick 30 seconds if you have any final thoughts about this.
[Sanjay Mehta]
Yeah, I mean, I do know the manual, kind of first approaches do lead to what we consider kind of experience hijacking, or stereotyping of users, which I think, today’s users are being trained on the power of individualization, due to their daily touchpoints. Whether it’s social network, media, Google, or Amazon. So, being more aligned to the expectation of the user from that, I think is very important. And then going back to, I think the idea of kind of macro influences of an experience, right? Which is why, or diagnosing why something is occurring is kind of the other side of AI that’s very compelling.
The ability to take all this macro data, inside your organization, and outside of your organization, to understand these trends. You know, there’s some things going on with the supplier, or even crawling social media, and looking at sentiment, and those sorts of things, are really powerful capabilities.
[Katie Florez]
Thank you, yeah. I wanna keep an eye on time, ’cause I know there are a lot of other sessions to attend. And in conclusion, I think two words come to mind, in summary of our debate. Better together. Human curation and machine learning coming together to provide truly connected and personal experiences for shoppers.
Thank you so much for joining us, Peter and Sanjay, and to all of our Activate attendees, thanks for being here. Sanjay and Peter will be attending sessions throughout the rest of the conference, and I hope you’ll say hello and ask any lingering questions. Have a wonderful rest of your day.