Five Ways Fusion Enhances the Value of a Hadoop Data Lake
Hadoop data lakes frustrate business leaders, data scientists, and analysts because more data means slower queries. See how machine learning helps you speed it up.
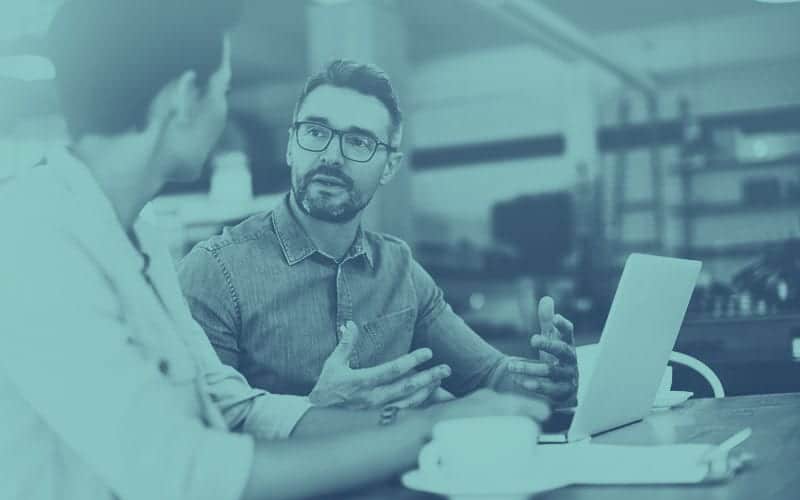
This is a very nostalgic blog post for me. I worked as a product marketer at Hortonworks from February 2013 to the summer of 2017. In those four-and-a-half years, I penned about 50 articles extolling the virtues of Hadoop and its surrounding cohort of projects from the Apache Software Foundation. (Don’t go looking for those posts. They didn’t survive the Cloudera-Hortonworks merger.)
Back then, we promoted the Apache Hadoop data lake as the best way to capture the value of big data, despite the “three V’s” of modern data: volume, velocity and variety. The value promise was sincere, and usually the first use case justified our customers’ investment in Hadoop, many times over.
There’s Gold In Them Thar Hills
But since the peak of the Hadoop gold rush in 2014-2015, some of the shine has faded. Pundits and practitioners have proudly proclaimed, “Hadoop is dead. Long live Hadoop.” In fact, you can find many blog posts or articles with variants of that title:
- 2019: “Hadoop is Dead. Long live Hadoop.” by Arun Murthy, a Hadoop founding father
- 2018: “Is Hadoop Officially Dead?” by Alex Woodie in Datanami
- 2016: “Hadoop is dead, long live Hadoop!” by Gartner’s Svetlana Sicular
- 2012: “Hadoop is dead. Long live Hadoop” by 451 Group‘s Matt Aslett
But even with all these doomsayers, Hadoop’s mortality continues lumbering along like a zombie for at least seven years. In September of this year, Cloudera announced quarterly revenue of nearly $200 million. If that’s considered dead, I hope I’m making that kind of money when I’m six feet under.
Any semi-objective analysis of the current landscape must reckon with the many thousands of petabytes still stored in production HDFS deployments all over the world.
So why all the doom and gloom?
This frustration and disillusionment comes from the difficulty extracting value from all that big, beautiful, multi-source data. It’s there – just within reach! -sitting in commodity servers. But we can’t use it the way we would like. Let’s blame that last mile problem on the “four S’s:” speed, science, sight, and socialization:
- Speed: Hadoop queries are notoriously slow.
- Science: Data scientists want to train ML models on lake data, but the feature engineering and data exploration required to implement these capabilities are slow, tedious, and technical.
- Sight: Visualization options for big data insights are neither beautiful nor interactive.
- Socialization: High priests and priestesses in Hadoop CoEs (Centers of Excellence) take in questions from the untrained masses and dole out answers as they work through the queue. Difficulty with self-service data discovery keeps insights from going viral.
The utopian vision of Hadoop augmenting the intelligence of millions of business and government employees as they visually interact with terabytes of data in real-time bliss…that hasn’t happened. Even though the data is continuously flooding into these data lakes, insight still comes in small drops.
Storage Doesn’t Automatically Mean Insights
This is because having the data under storage is not the same as having the data under insight.
But happy days are here again! Lucidworks Fusion makes big data easily accessible with AI-powered search and a method of interaction that everyone already knows how to use: the search box. Now everyone across the organization can search big data, without having to learn specialized tools like Hive, Spark, HBase, or Kafka. Anyone can find insights as quickly as they can type a search query or click on a facet as they browse.
5 Reasons Lucidworks Fusion Improves Your Hadoop ROI
1. Deliver Faster Queries and Better Answers
Analysts and data scientists have become accustomed to writing queries and waiting minutes for the results to return. They have accepted this because slow insights are better than no insights.
Fusion takes the waiting out of Hadoop data exploration. Results for natural language queries return immediately, and Fusion supports thousands of queries per second.
2. Let Everyone Access Insights
Data lakes have their de facto gatekeepers to the insights they contain. If you can’t use Hive, Impala, or another Big Data access engine, you need one of the chosen ones to ask the questions for you.
Fusion frees anyone to explore the data lake by asking natural language questions in more than 60 languages. Or they can use a Fusion SQL service that lets organizations leverage their existing investments in BI tools by using JDBC and SQL to analyze data managed by Fusion.
3. Operationalize Machine Learning
Organizations want to adopt machine learning (ML) to make operations more efficient, but ML projects fail frequently because executives, data scientists, and the DevOps team find collaboration difficult.
Fusion comes with advanced ML models. Plus, any existing Python models can be easily integrated, or data scientists can publish new models to Fusion pipelines. Users generate a constant flow of signals that train those models. Proactive recommendations for ecommerce merchandising, next best investment decisions or legal e-discovery (to name just a few) become more predictive with each search, click or download.
4. Don’t Move Data, Just Connect with APIs
It was difficult getting so much diverse data into your data lake. You shouldn’t have to move it again. Apache Solr, at the core of Fusion, has been searching distributed data in the Hadoop Distributed File System (HDFS) for over a decade.
When I was at Hortonworks, we partnered with Lucidworks to support native Apache Solr, before Lucidworks built Fusion. Lucidworks open-sourced six connectors for indexing content from Hadoop to Solr, for: HDFS, Hive, Pig, HBase, Storm, and Spark. Search the data where it lives.
5. Don’t Worry. It’s Secure.
Thirty percent of the US Fortune 100 use Lucidworks Fusion in production deployments every day. The platform comes with multiple options for authenticating and authorizing users, including Active Directory and Kerberos. Fusion can restrict access to any resource and also supports “security trimming” for many repositories like Google Drive and Microsoft Sharepoint, applying access control rights inherited at ingestion.
So if you’ve invested in a Hadoop data lake and the business value is blocked, talk to us about adding Fusion to your ecosystem. Hadoop ain’t dead, it just needs some AI-powered search, powered at its core by open-source cousins of Hadoop: Apache Solr and Apache Spark.
LEARN MORE
Contact us today to learn how Lucidworks can help your team create powerful search and discovery applications for your customers and employees.