Enhancing Search Experiences: Key Developments in Search Data Science (Part 1)
The essential, expanding role of AI in modern search experiences.
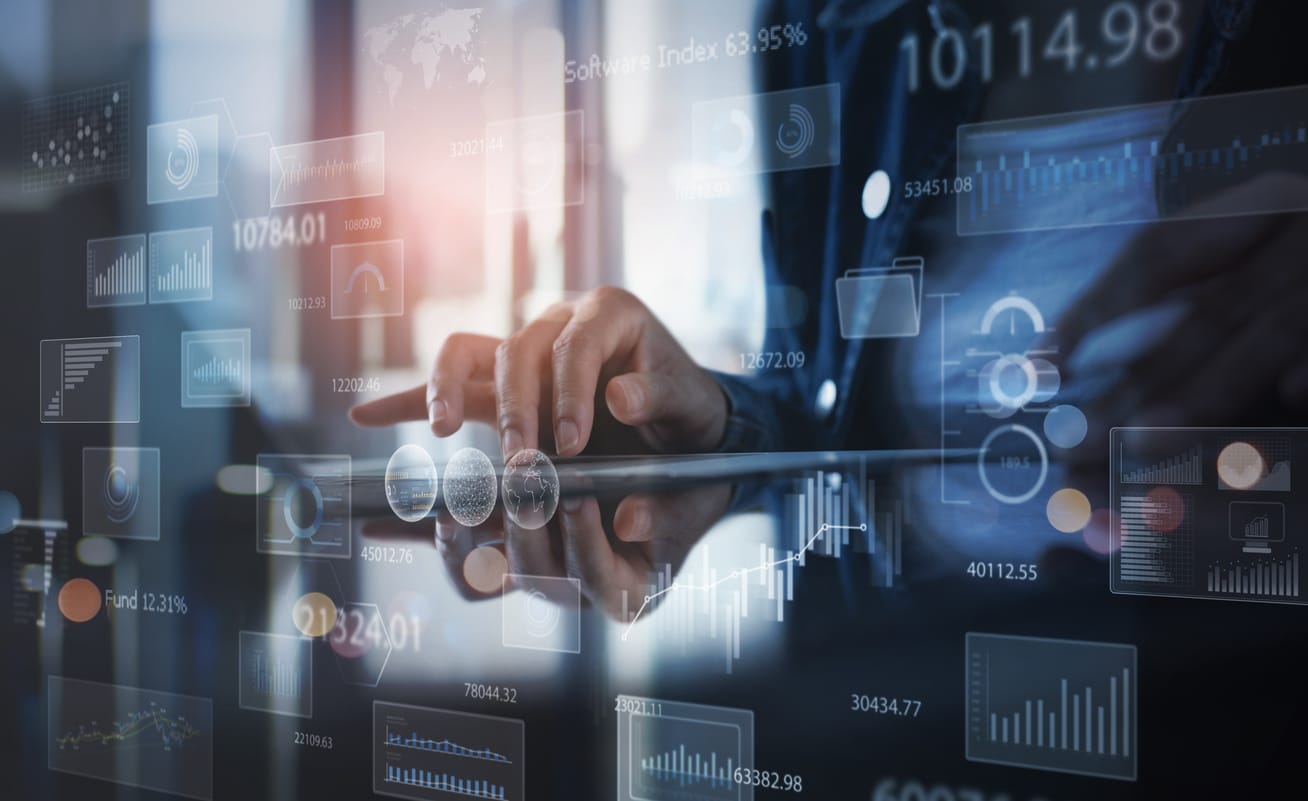
Search technology has come a long way in a few short years. Today, it encompasses incredibly sophisticated platforms that combine large language models (LLMs), semantic search methodologies, generative AI, and other advanced technologies to create entirely new search experiences.
Of course, given all the advanced math and science that powers these solutions, it can be challenging for non-data scientists to keep up. And it can be even more difficult to understand exactly how these complex and abstract concepts translate into business benefits — like better customer experiences, increased efficiency, and new sources of revenue.
To help you strengthen the connections between the latest search data science and the practical business benefits it can offer, here’s a two-part article that explores a few important search technology trends and concepts you should be aware of. Part 1 explores key AI-related issues shaping modern search experiences. Then, Part 2 dives deeper into the AI-driven evolution of search experiences from basic queries to natural conversations.
Combining semantic search with traditional query matching for better search experiences
Semantic search is a relatively new methodology that leverages deep learning models to perform searches based on meaning and intent rather than keyword matches. It has quickly become a driving force in the search industry — and for good reason. Semantic search technology contributes to more personalized and relevant search experiences, and it can virtually eliminate the dreaded “no results” searches that often cause customers to abandon retail websites.
However, it’s important to understand that semantic search technologies work best when they complement — rather than replace — more traditional query match searching. Semantic searches are ideal for multi-word queries, conceptual searches, natural language questions, and other complex interactions. But basic keyword searches can still deliver faster, more accurate results for single-word, exact-match queries. And most shoppers still depend on these types of simple keyword queries when they’re looking for a specific item or brand.
Fortunately, this is a clear case where you don’t have to choose. Many organizations are embracing a hybrid approach that integrates a keyword search engine with a semantic vector search engine on a single platform. If they’re designed correctly, these hybrid search solutions can combine the advanced intent and meaning-based capabilities of semantic search with the speed and simplicity of query matching into one seamless search experience.
The challenges of scaling AI-powered search technology
Large language models (LLMs), semantic vector search engines, and other new AI-powered search technologies are undeniably powerful. They also require an enormous amount of data and computing resources — especially as they scale up to meet the demands of large organizations. In practical terms, this can translate into unacceptably slow response times and spiraling costs.
Data scientists and researchers are actively tackling this challenge throughout the AI-driven search landscape. They’re doing so by developing and fine-tuning smaller, targeted AI models that demand fewer computing resources. Additionally, they’re building specialized cloud-based search platforms that efficiently use shared computing power at an affordable rate. Moreover, they’re exploring hybrid search platforms capable of assigning certain search tasks to quicker, less compute-intensive query-based engines.
Search professionals should prioritize understanding the potential effects on performance and cost caused by current advanced AI-powered search technologies. They should focus on seeking partnerships, platforms, and solutions geared toward making cutting-edge search science both practical and cost-effective for real businesses.
The risks of AI model bias and impacts on search experiences
All too often, AI models unintentionally capture and reflect the biases of the data used to train them. In the search world, these biased models and data can have a significant impact on the accuracy of search recommendations and results, and it can also open the door to new regulatory risks.
As AI becomes a more pervasive component of search experiences, search professionals must recognize the potential biases, understand how to spot them, and swiftly develop effective strategies to address them. This involves selecting search partners and platforms dedicated to eliminating model and data bias, offering transparency and insight into the AI models embedded in their solutions.
Next up: The data science driving the shift from queries to conversations
Part 2 of this discussion explores how AI is transforming search experiences into natural, extended conversations. In the meantime, learn more about Lucidworks AI solutions.
Best of the Month. Straight to Your Inbox!
Dive into the best content with our monthly Roundup Newsletter!
Each month, we handpick the top stories, insights, and updates to keep you in the know.