Enhance Product Discovery with AI-Powered Recommenders
Learn how AI-powered recommenders put the right products and content in front of your customers, with just the right amount of human touch.
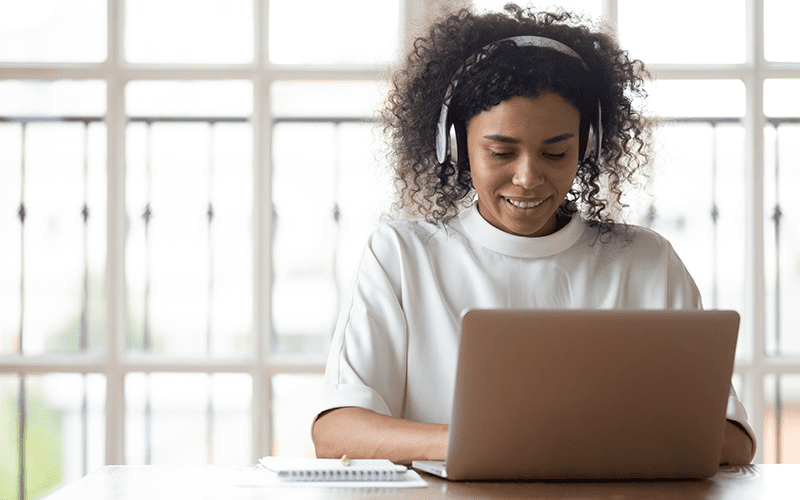
Delivering the best possible shopping experience is every retailer’s dream and providing shoppers with relevant, compelling recommendations is key.
Let’s say Greg’s family got a puppy during the pandemic. As they plan on bringing the puppy home from the shelter, Greg goes online to buy the standard collection of things you’re going to need for their new canine family member: toys, food, dishes, brushes, treats, and a crate. The shelter tells Greg that the new dog has a gluten sensitivity so he’ll need to feed her only grain-free dog foods.
Greg goes online to his favorite pet retailer to shop for dog food for his new furry friend. He goes to the dog food category and checks the filter to only see grain-free foods. Lots of options:
Like most people he goes for the first result first, a grain-free turkey-based dry food:
Product description makes sense. This is what he’s looking for. But it’s a little pricey. Greg wants to see other options and notices the Customers Also Viewed bloc further down the page:
Hmmm. These aren’t very good recommendations. Melting ice isn’t a problem since Greg lives in Florida, and that’s not even a food! There’s a whole grain product also listed – and also irrelevant. The other three options are grain-free. In this case, two of the five recommended products don’t apply at all to what he is shopping for. Greg goes with that initial option and completes his transaction. The toys and food are on the way.
Later while posting puppy photos to Facebook, Greg sees a retargeted ad from that same pet retailer promoting some more related dog food products.
But only two of these are grain free. Another missed opportunity!
The next day, Greg gets a promotional email from the retailer thanking him for his order and again including a couple more product recommendations.
And they have nothing to do with his new puppy who doesn’t have a sensitive stomach and isn’t a large dog (yet).
Greg has now seen several sets of recommendations across several marketing channels from the same retailer. Each placement was an opportunity to increase engagement and drive conversions. But these opportunities can be missed due to weak, irrelevant recommendations.
Let’s look at another example:
Greg has been working at home the past year and decides if offices aren’t opening any time soon, he might as well get some comfy house slippers to wear around the house. He goes to the site for his favorite online shoe store and looks for slippers:
He clicks on the one in the lower left and the product detail page displays:
Okay great – looking good – these might be the ones he wants. But there’s a recommendations block further down the page:
These are all women’s shoes which is distinctly not what he is shopping for. Another missed opportunity to increase average order value and conversion.
Retailers have opportunities to have their recommenders churning out product suggestions to customers at all phases of the sales journey and for good reason. Recommenders work.
Recommendations Raise Average Over Value and Conversions
A recent study found that as engagement with recommendations goes up, AOV goes off the charts:
That’s a lift of 370% just from one extra click, climbing up to about five clicks. The same study saw a huge boost in the conversion rates as well:
Implementing and properly tuning recommenders can be a key way for retailers to raise AOV and conversion rates and keep customers coming back and buying more.
Let’s look at a few ways to do this:
“Self-Driving Mode”
One approach is completely machine-based, like a self-driving car. The algorithm is looking forward and farther down the road, it’s seeing what’s in the rear view mirror along with what’s immediately around it. Content-based recommenders often follow this approach for providing high quality item-to-item recommendations. This approach is especially useful when you have a new site with no signals or user behavior data.
Another machine-only approach is to surface popular and trending items and categories. This can be used to surface both related products and related content like branded content and other documents the customer might find useful in their shopping experience.
Human and AI Synergies
Adding in some human intervention, we get to recommenders that run for most part by themselves but there is some manual work going on. This is where popular algorithms like Bayesian personalized ranking (BPR) and alternating least squares (ALS) are put to work. Semantic vector search is also used a lot in these examples. Semantic vector search looks at the relationships to products between each other and across the whole catalog.
An real-life analogy would be going to your supermarket to get some shellfish to cook for dinner. At the fish counter are items you might include in a seafood meal like lemons and tartar sauce. Lemons are officially in bins over in the produce section and the tartar sauce is over in the condiments aisle. But by finding how products cluster and get bought in groups, you can find that relationship. You are better understanding user intent and what the goal of their shopping visit is.
In addition to how products are bought together, this approach also uses typical user signals to enhance these recommendations: queries, clicks, add to cart, purchases, support tickets, and the like.
Merchandisers Still Know Best
And sometimes you still need the human touch and expertise to get your recommenders to be fully optimized. Ideally merchandisers can put in business rules for boosting, blocking, and pinning products in any given assortment. So owners of grain-free dogs see those food choices and men shopping for shoes see men’s house slippers. Another example would be using color to define recommendations. When a shopper is looking at women’s red jackets, the recommendations could include red items beyond coats and jackets.
The easier it is to put these rules in place, test and measure them, and make adjustments the easier it is for retailers to act quickly and decisively in reacting to shopping trends and customer tastes. Our Predictive Merchandiser gives merchandisers and admins drag-and-drop control over this whole process.
Things Are Changing Fast
All of the above types of recommenders have focused on what the customer encounters on their shopping journey. But this technology can also be put to good use with online concierge style interactions or in-store engagements with sales associates. The employee can see recommendations for a particular customer and offer their own take or opinion as they walk the customer through each one.
This article was based on Garrett Schwegler’s session “AI-Powered Recommenders: Self-Driving Mode and Human Intervention” from our Activate Product Discovery 2021 event. Looking for more information on how recommenders and merchandisers can play together? Read this blog next: “Give Merchandisers Control of Landing Pages to Curate Shopper Experience.”
LEARN MORE
Contact us today to learn how Lucidworks can help your team create powerful search and discovery applications for your customers and employees.